Birdseye raises $3.5M in seed funding to revolutionize how brands target customers
Make customer data your QSR's secret sauce
Make customer data your QSR's secret sauce
BirdseyeQSR empowers quick service restaurants with AI-powered analytics and personalization solutions. Whether your goal is to increase customer loyalty, drive more visits, or maximize revenue, we deliver the insights and tools needed to achieve real results.
BirdseyeQSR empowers quick service restaurants with AI-powered analytics and personalization solutions. Whether your goal is to increase customer loyalty, drive more visits, or maximize revenue, we deliver the insights and tools needed to achieve real results.
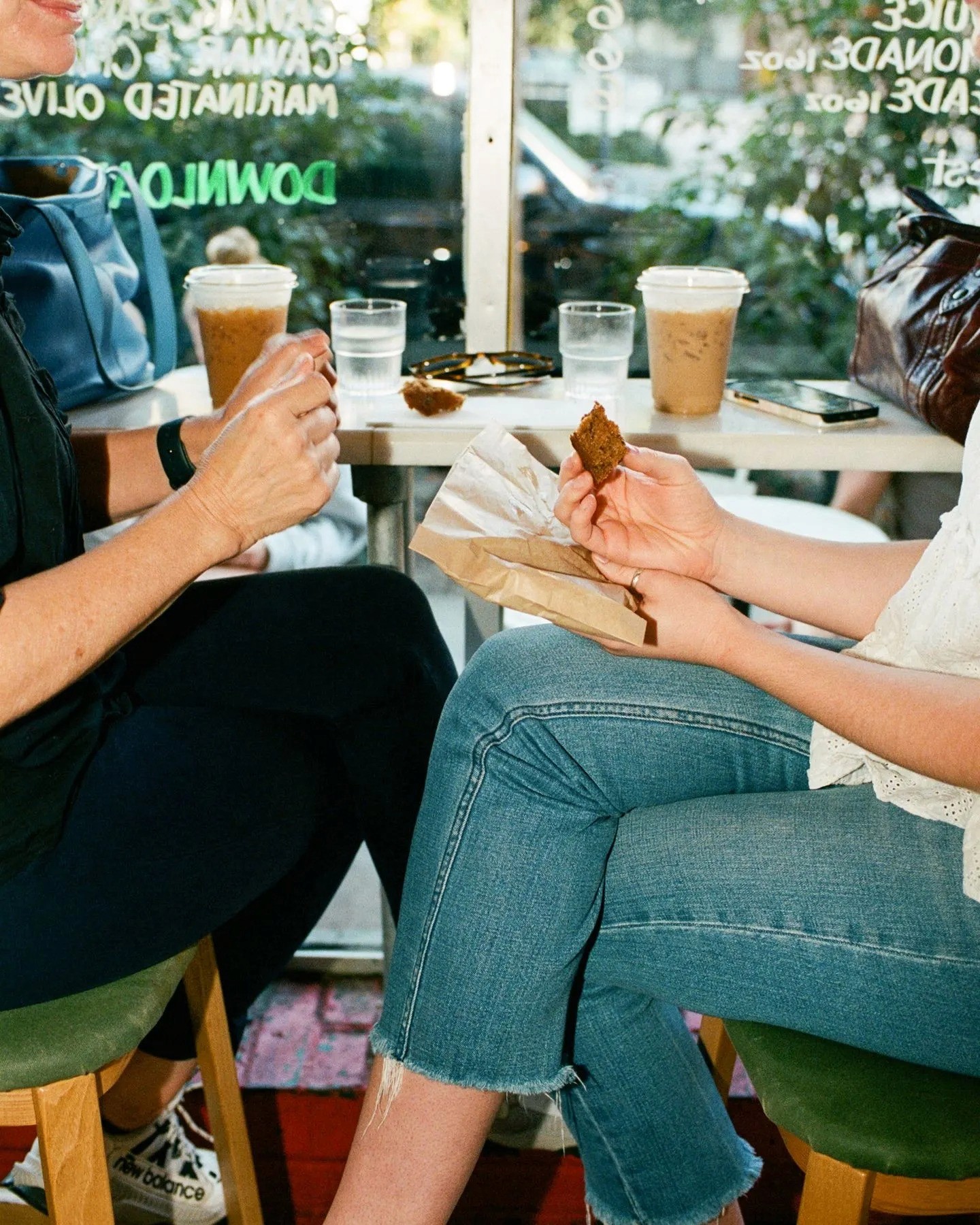
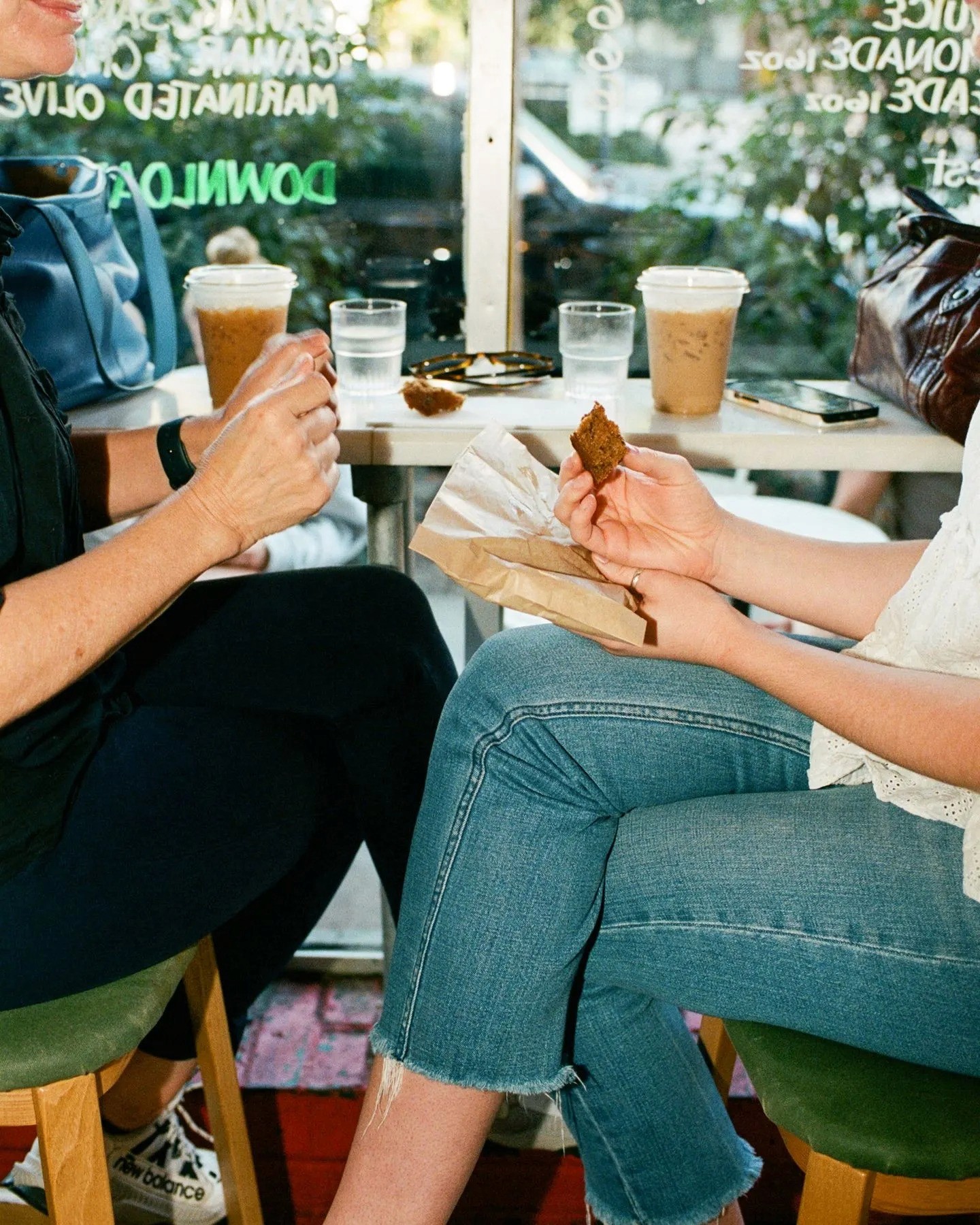
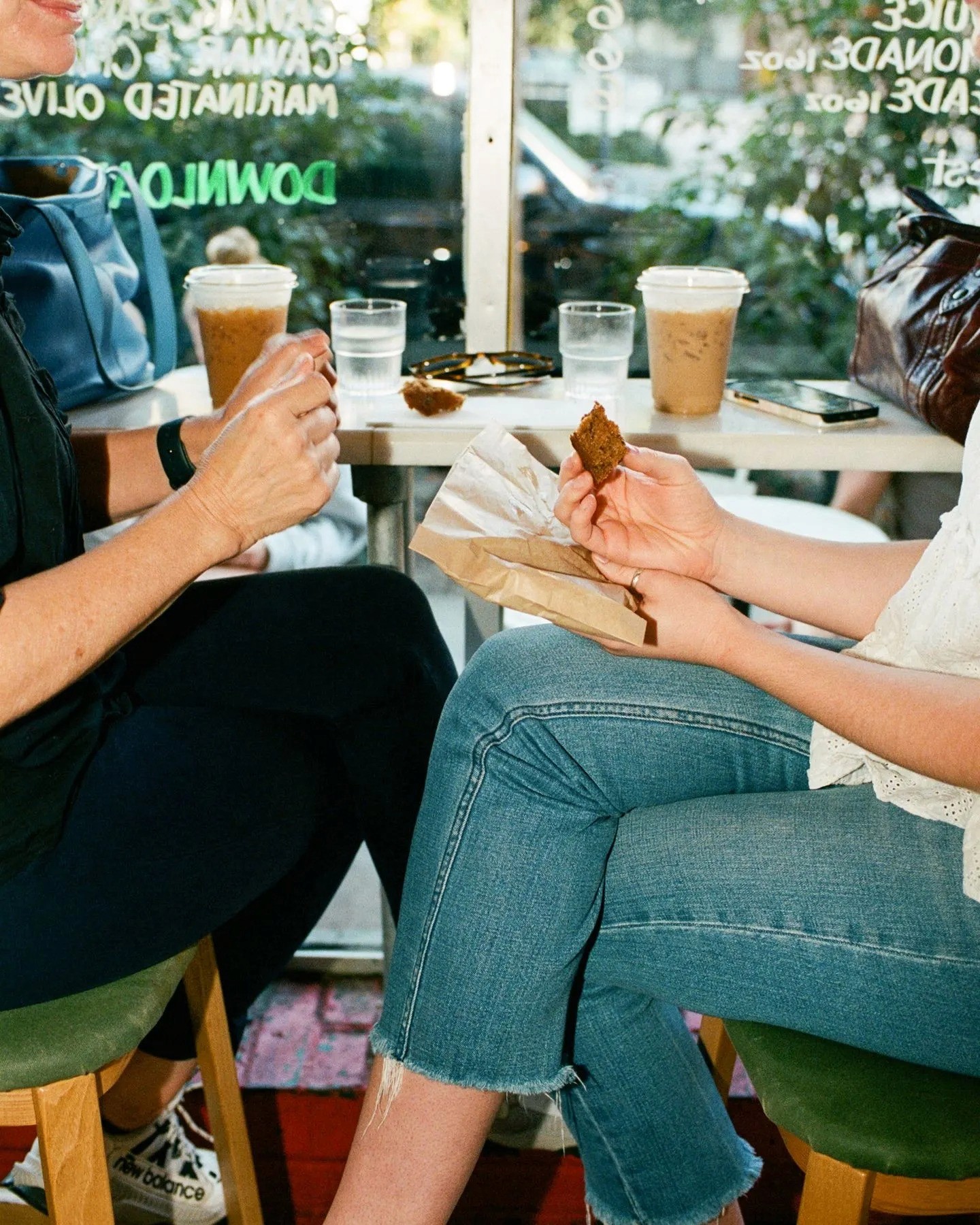
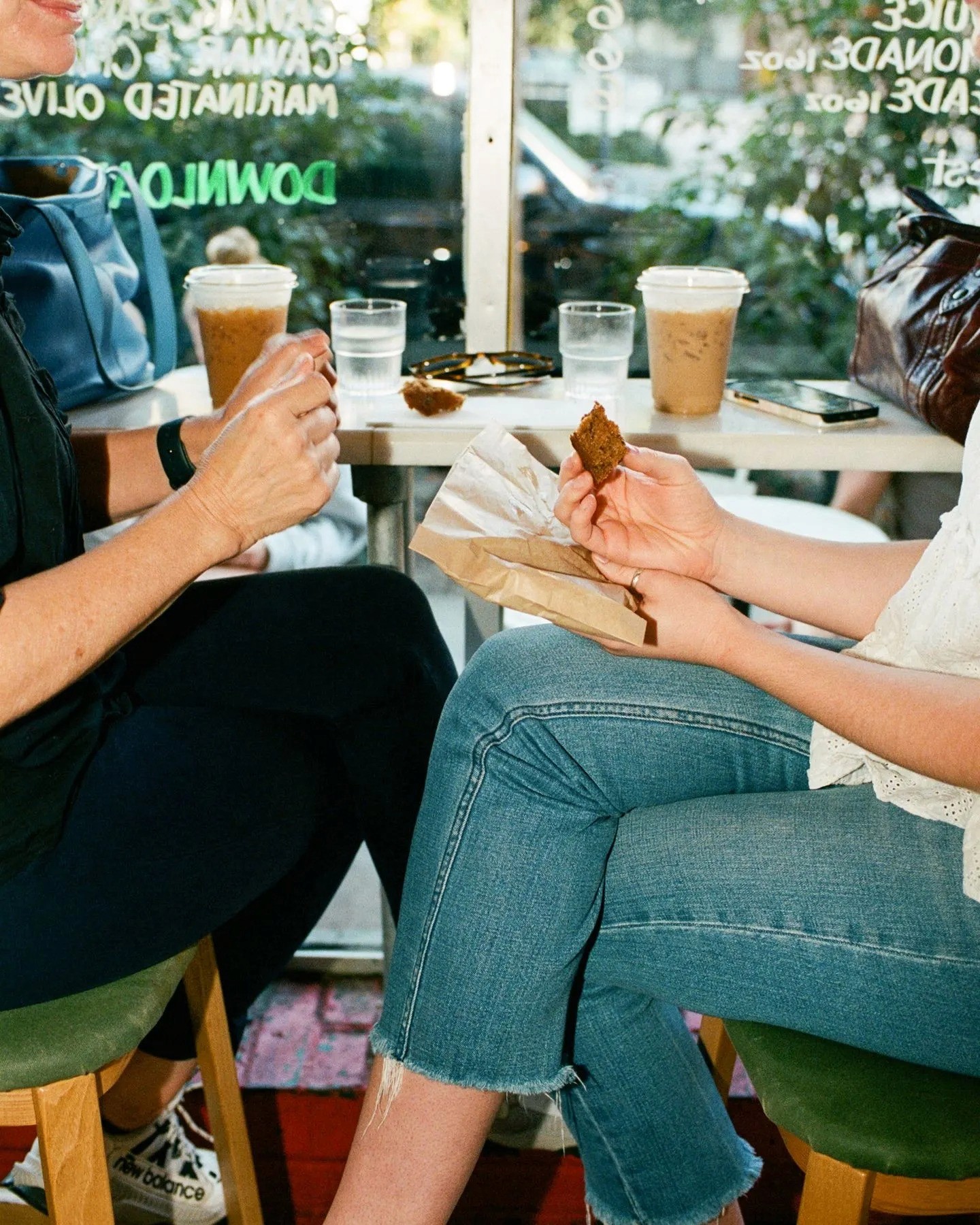
Deliver hyper-personalized customer experiences
Deliver hyper-personalized customer experiences
Birdseye delivers cutting-edge Advanced Propensity Modelling to help QSRs effectively engage customers, drive sales, and increase customer lifetime value (CLV).
Personalized Offers
AI-powered models serve up personalized offers across touch points based on diners' past behavior, boosting conversions without relying on heavy discounts.
Personalized Offers
AI-powered models serve up personalized offers across touch points based on diners' past behavior, boosting conversions without relying on heavy discounts.
Personalized Offers
AI-powered models serve up personalized offers across touch points based on diners' past behavior, boosting conversions without relying on heavy discounts.
Actionable Insights
Birdseye analyzes key customer behaviors—recency, frequency, spend, and taste preferences. Some like it grilled, others go for fried—we take out the guesswork.
Actionable Insights
Birdseye analyzes key customer behaviors—recency, frequency, spend, and taste preferences. Some like it grilled, others go for fried—we take out the guesswork.
Actionable Insights
Birdseye analyzes key customer behaviors—recency, frequency, spend, and taste preferences. Some like it grilled, others go for fried—we take out the guesswork.
Customer Clustering
Segment your audience to target high-value customers with precision. Tailored offers increase engagement, loyalty, and ROI, driving sustainable growth and long-term success for your business.
Customer Clustering
Segment your audience to target high-value customers with precision. Tailored offers increase engagement, loyalty, and ROI, driving sustainable growth and long-term success for your business.
Customer Clustering
Segment your audience to target high-value customers with precision. Tailored offers increase engagement, loyalty, and ROI, driving sustainable growth and long-term success for your business.
Ongoing Optimization
Birdseye’s AI continuously learns from diner interactions and preferences, optimizing future campaigns to boost engagement and drive higher order values.
Ongoing Optimization
Birdseye’s AI continuously learns from diner interactions and preferences, optimizing future campaigns to boost engagement and drive higher order values.
Ongoing Optimization
Birdseye’s AI continuously learns from diner interactions and preferences, optimizing future campaigns to boost engagement and drive higher order values.
Data Integration
Birdseye integrates loyalty, sales, and third-party data via SFTP or API, combining sources like gift card sales and transactions to deliver deep analytics and personalized offers for QSRs.
Data Integration
Birdseye integrates loyalty, sales, and third-party data via SFTP or API, combining sources like gift card sales and transactions to deliver deep analytics and personalized offers for QSRs.
Data Integration
Birdseye integrates loyalty, sales, and third-party data via SFTP or API, combining sources like gift card sales and transactions to deliver deep analytics and personalized offers for QSRs.
Data Security & Protection
Birdseye will work directly within your environment and infrastructure (servers, cloud, etc.), ensuring full ownership of all data, models, and work stays solely with you.
Data Security & Protection
Birdseye will work directly within your environment and infrastructure (servers, cloud, etc.), ensuring full ownership of all data, models, and work stays solely with you.
Data Security & Protection
Birdseye will work directly within your environment and infrastructure (servers, cloud, etc.), ensuring full ownership of all data, models, and work stays solely with you.
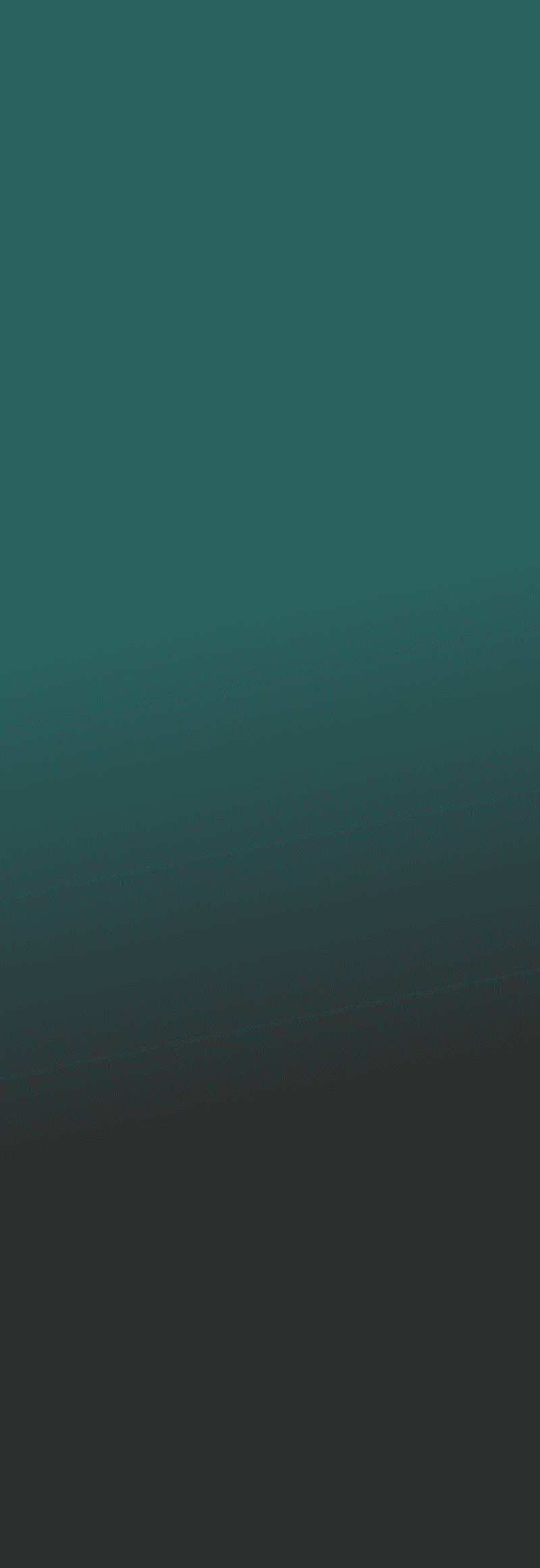
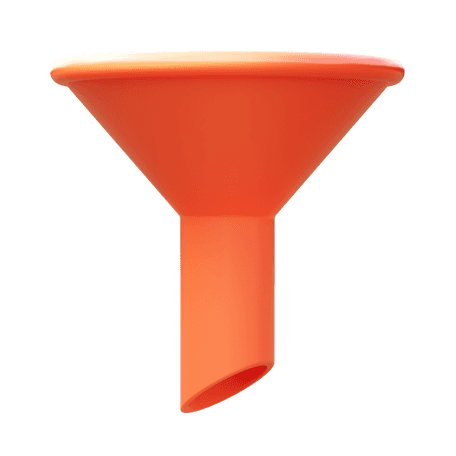
Birdseye organizes your transactional data for clarity
Birdseye analyzes purchase behavior to identify each customer's favorite menu items and offers.
Customers receive personalized offers tailored to their preferences, boosting loyalty and satisfaction.
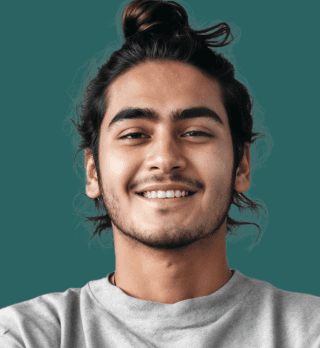
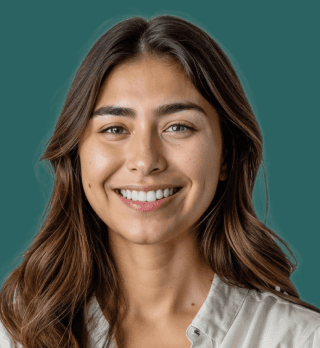
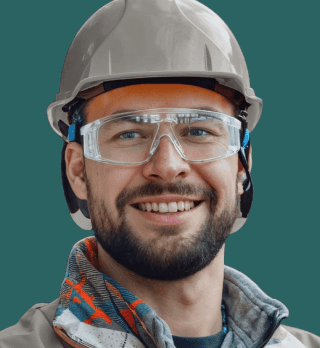
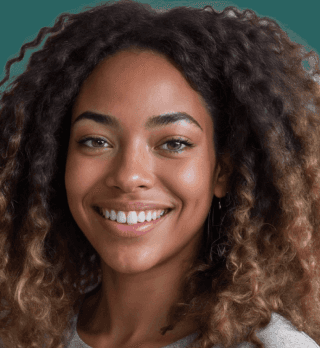
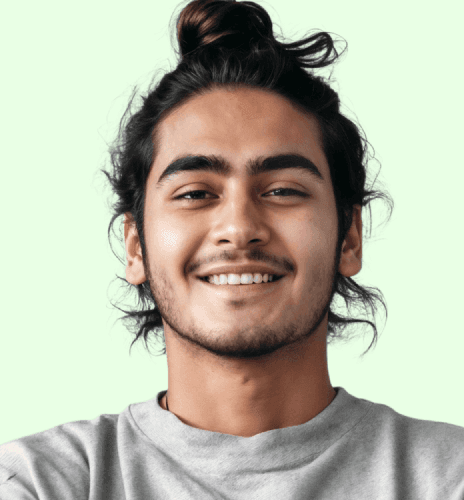
Preferences
Spicy
Takeout
Discount Sensitivity
Low
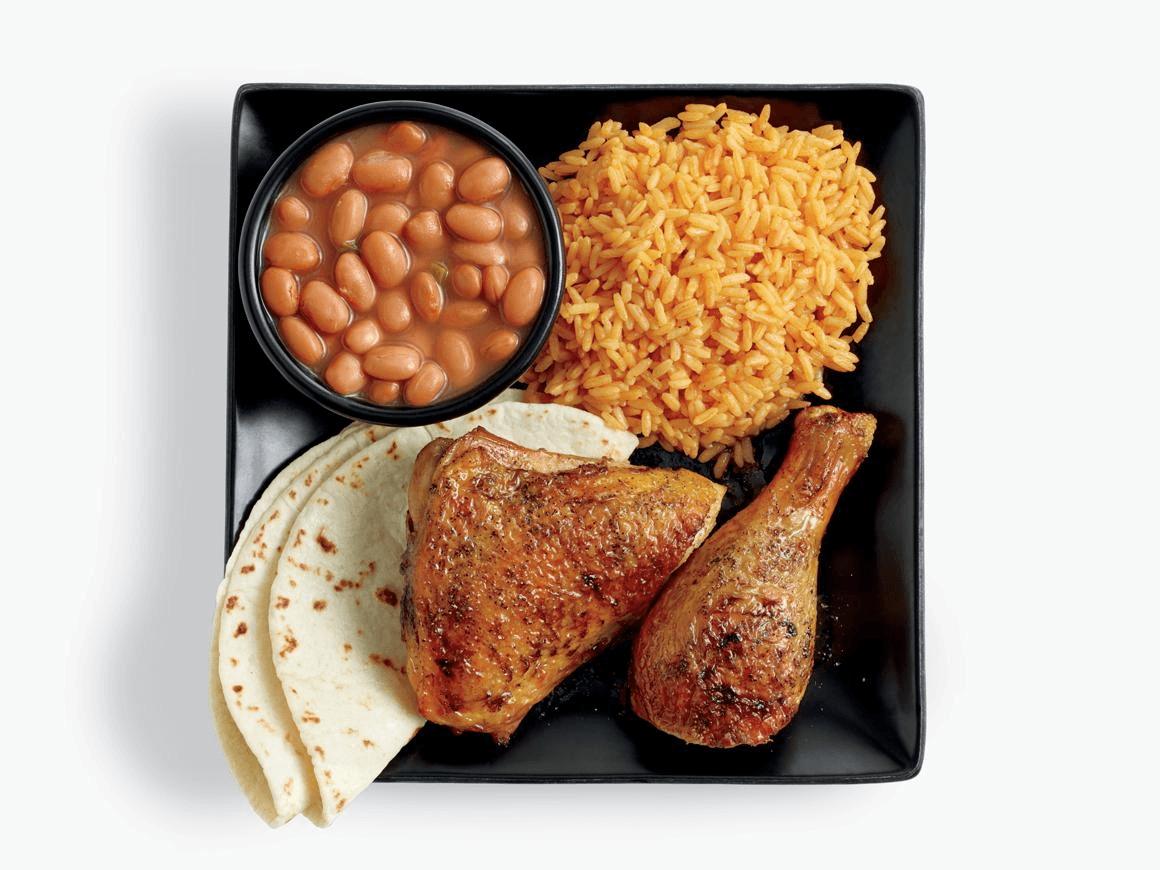
Craving Something Tasty?
Hey Diego,
Get 10% Off Your Takeout Order! Enjoy our
fire-grilled chicken with your limited time offer.
SPI_Diego342
Order now
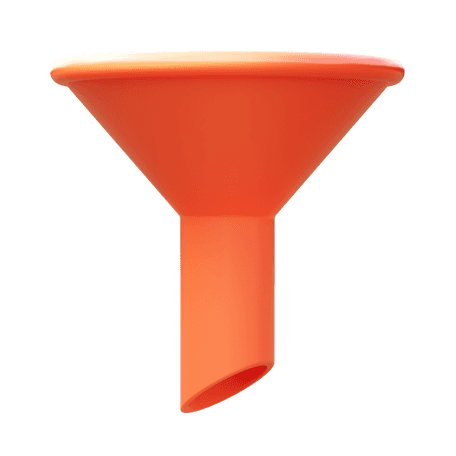
Birdseye organizes your transactional data for clarity
Birdseye analyzes purchase behavior to identify each customer's favorite menu items and offers.
Customers receive personalized offers tailored to their preferences, boosting loyalty and satisfaction.
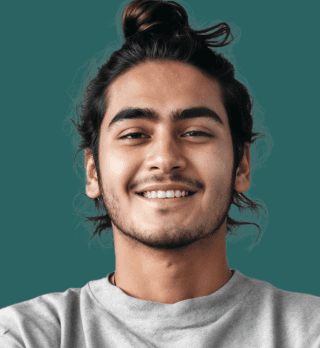
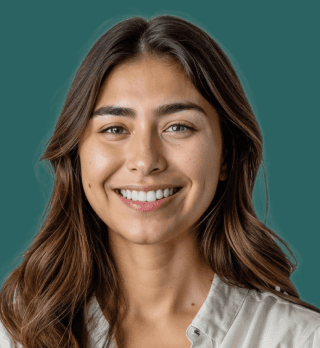
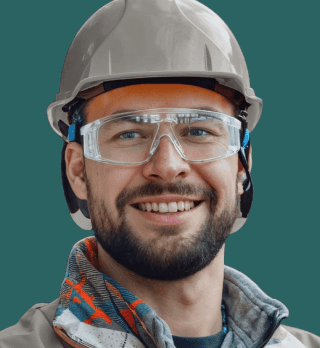
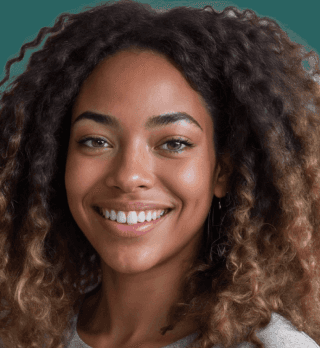
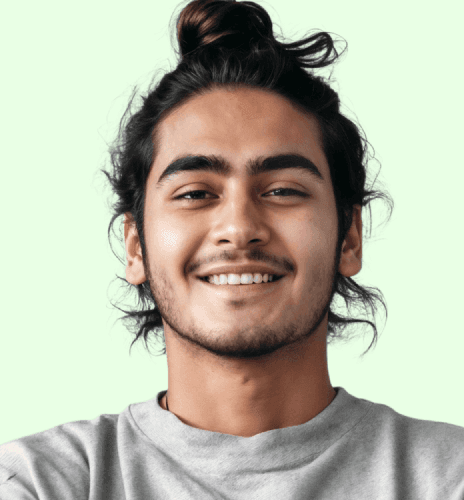
Preferences
Spicy
Takeout
Discount Sensitivity
Low
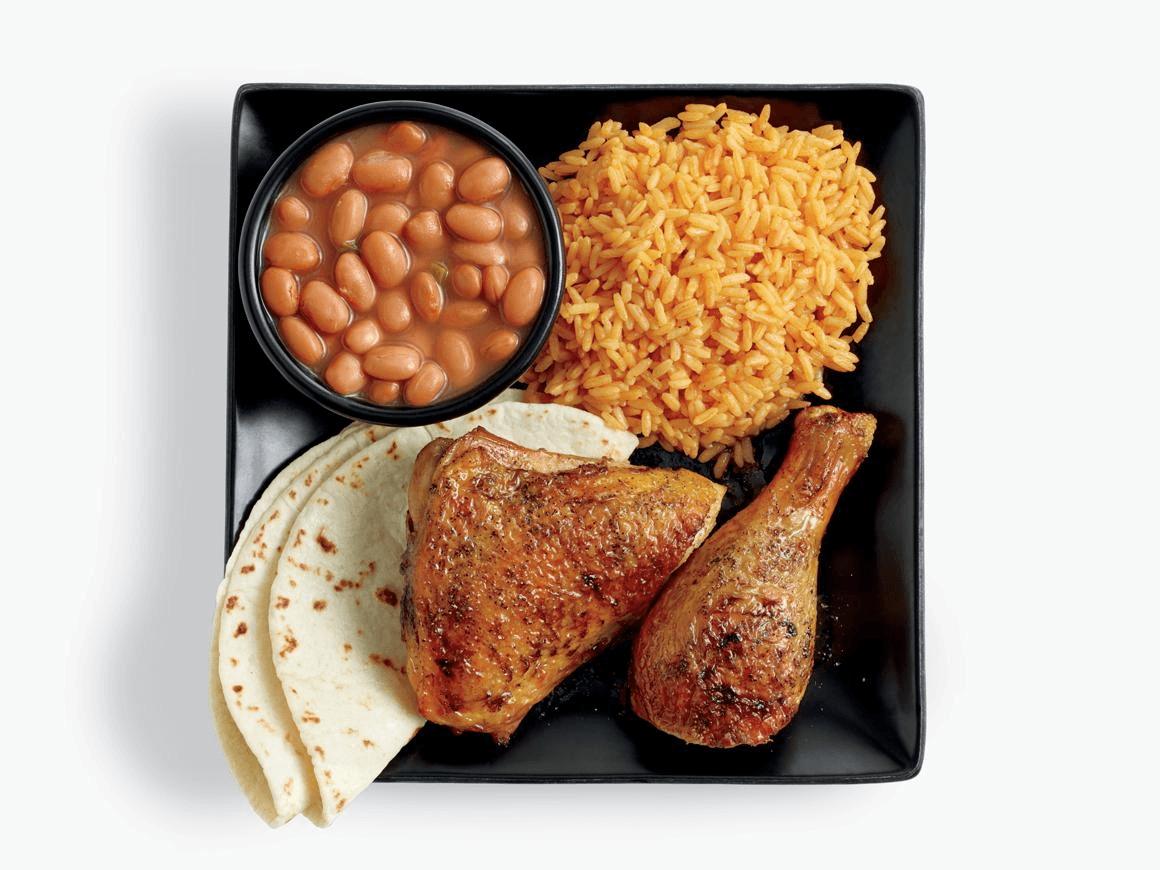
Craving Something Tasty?
Hey Diego,
Get 10% Off Your Takeout Order! Enjoy our
fire-grilled chicken with your limited time offer.
SPI_Diego342
Order now
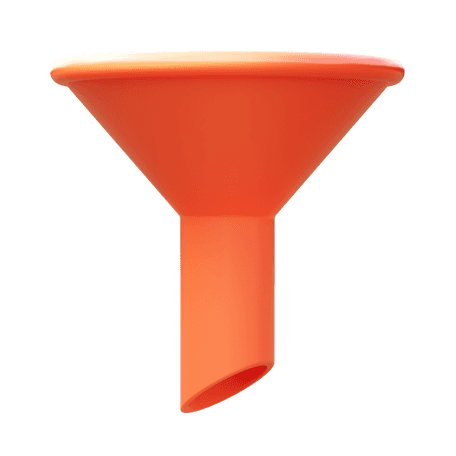
Birdseye organizes your transactional data for clarity
Birdseye analyzes purchase behavior to identify each customer's favorite menu items and offers.
Customers receive personalized offers tailored to their preferences, boosting loyalty and satisfaction.
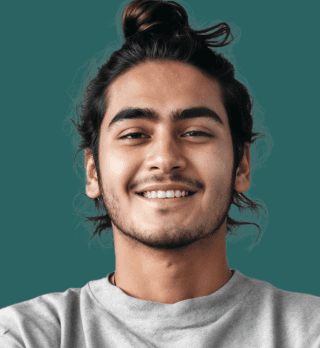
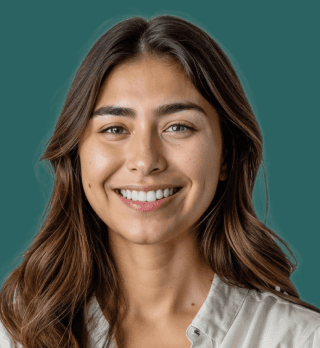
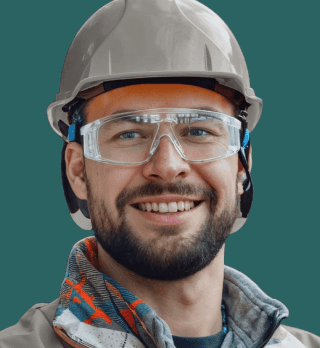
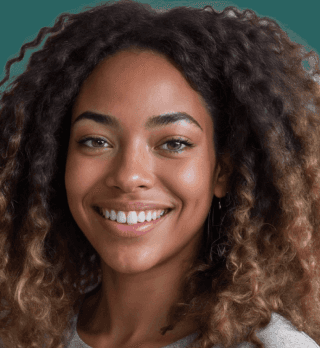
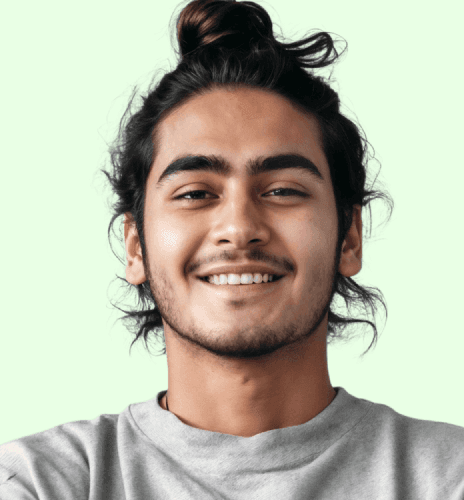
Preferences
Spicy
Takeout
Discount Sensitivity
Low
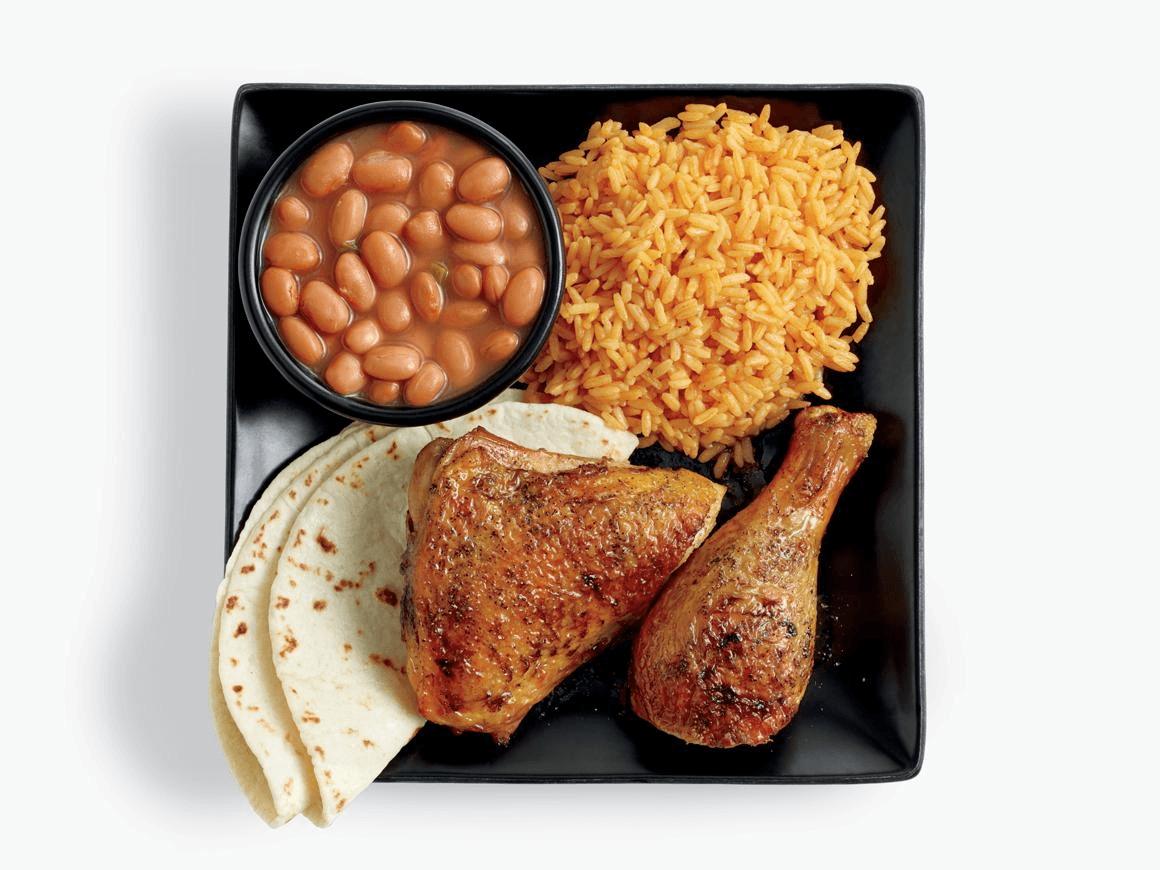
Craving Something Tasty?
Hey Diego,
Get 10% Off Your Takeout Order! Enjoy our
fire-grilled chicken with your limited time offer.
SPI_Diego342
Order now
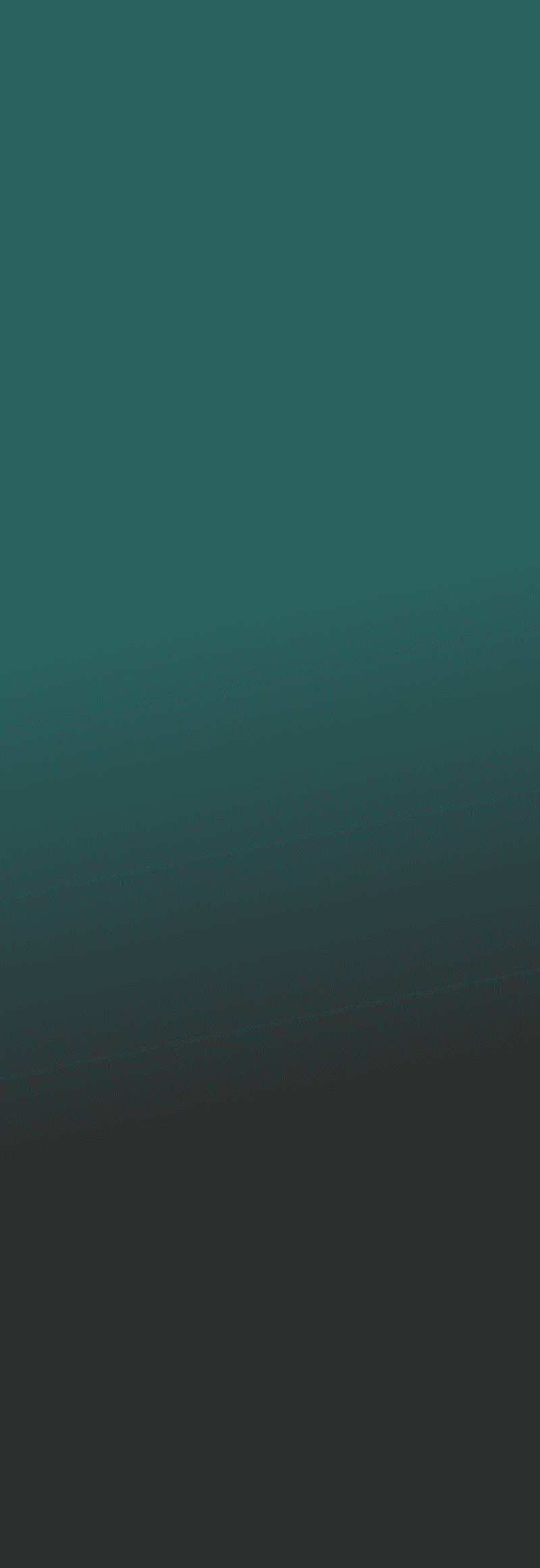
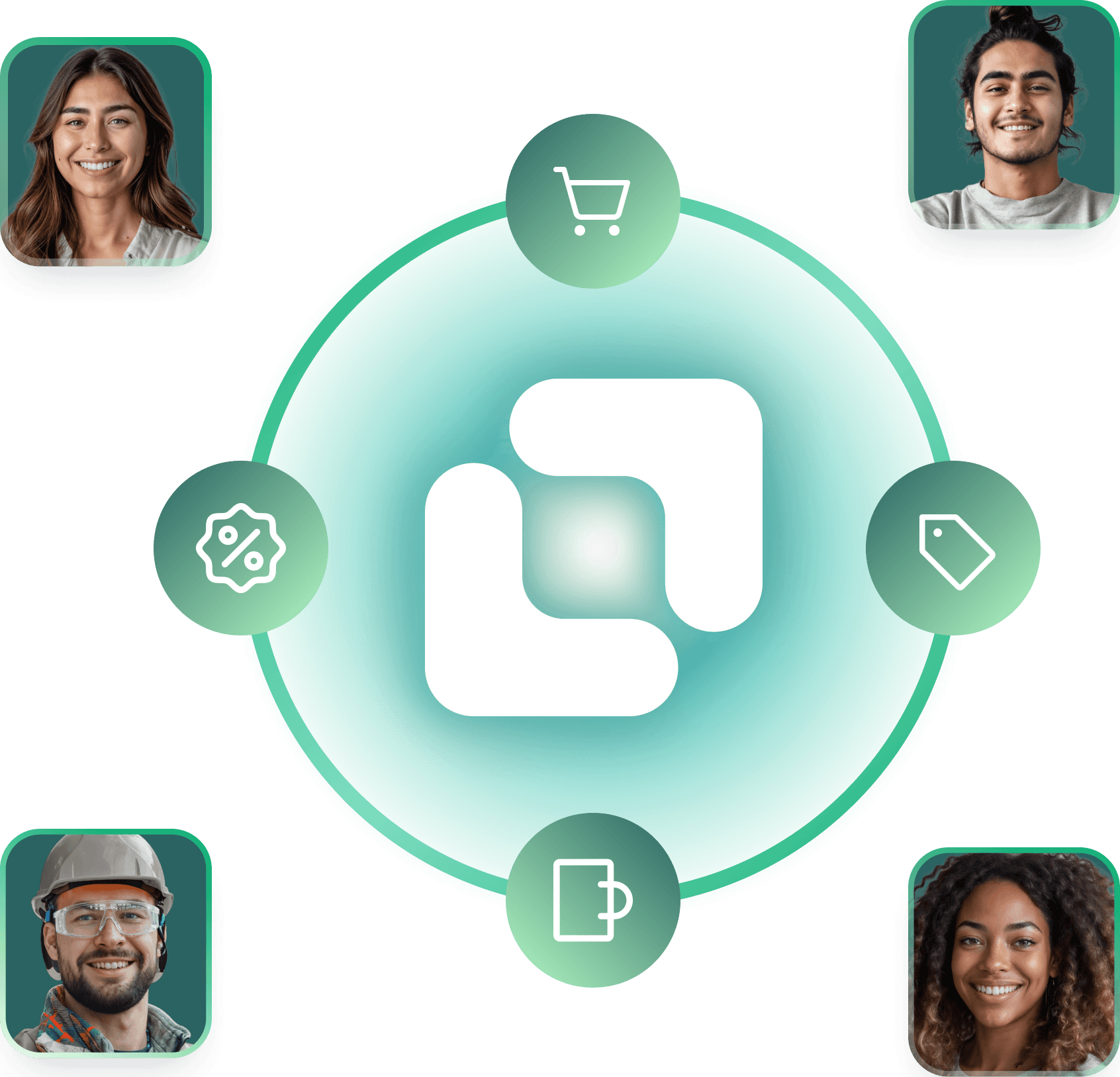
Birdseye organizes your transactional data for clarity
Birdseye analyzes purchase behavior to identify each customer's favorite menu items and offers.
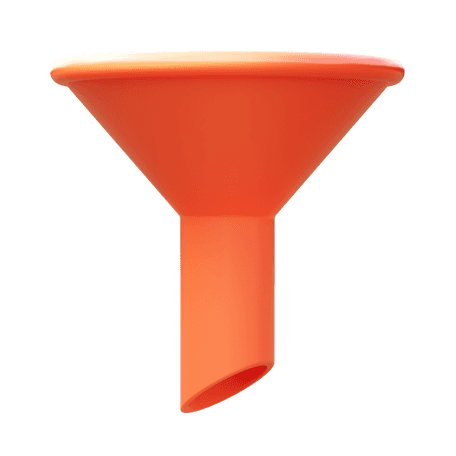
Customers receive personalized offers tailored to their preferences, boosting loyalty and satisfaction.
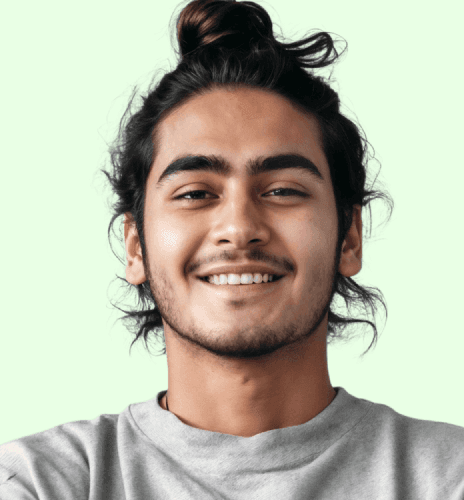
Preferences
Spicy
Takeout
Discount Sensitivity
Low
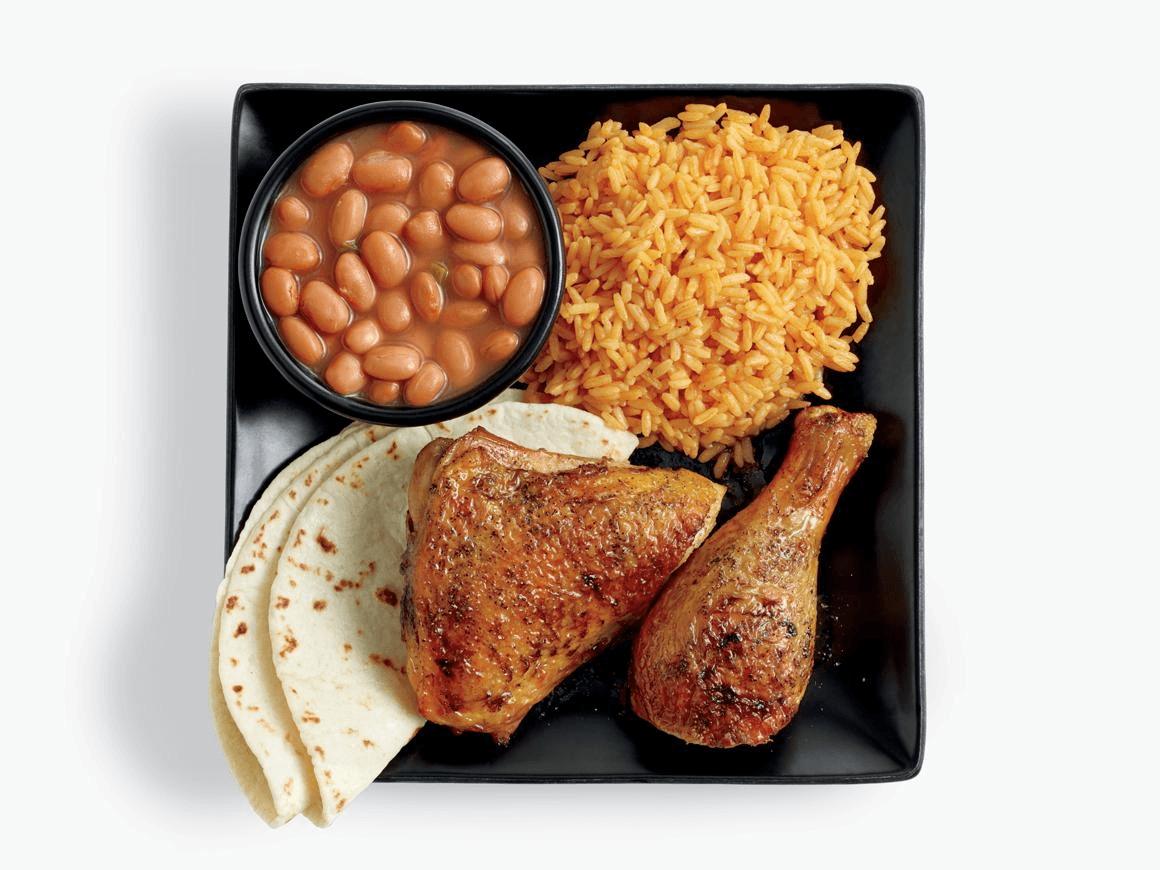
Craving Something Tasty?
Hey Diego,
Get 10% Off Your Takeout Order! Enjoy our
fire-grilled chicken with your limited time offer.
SPI_Diego342
Order now
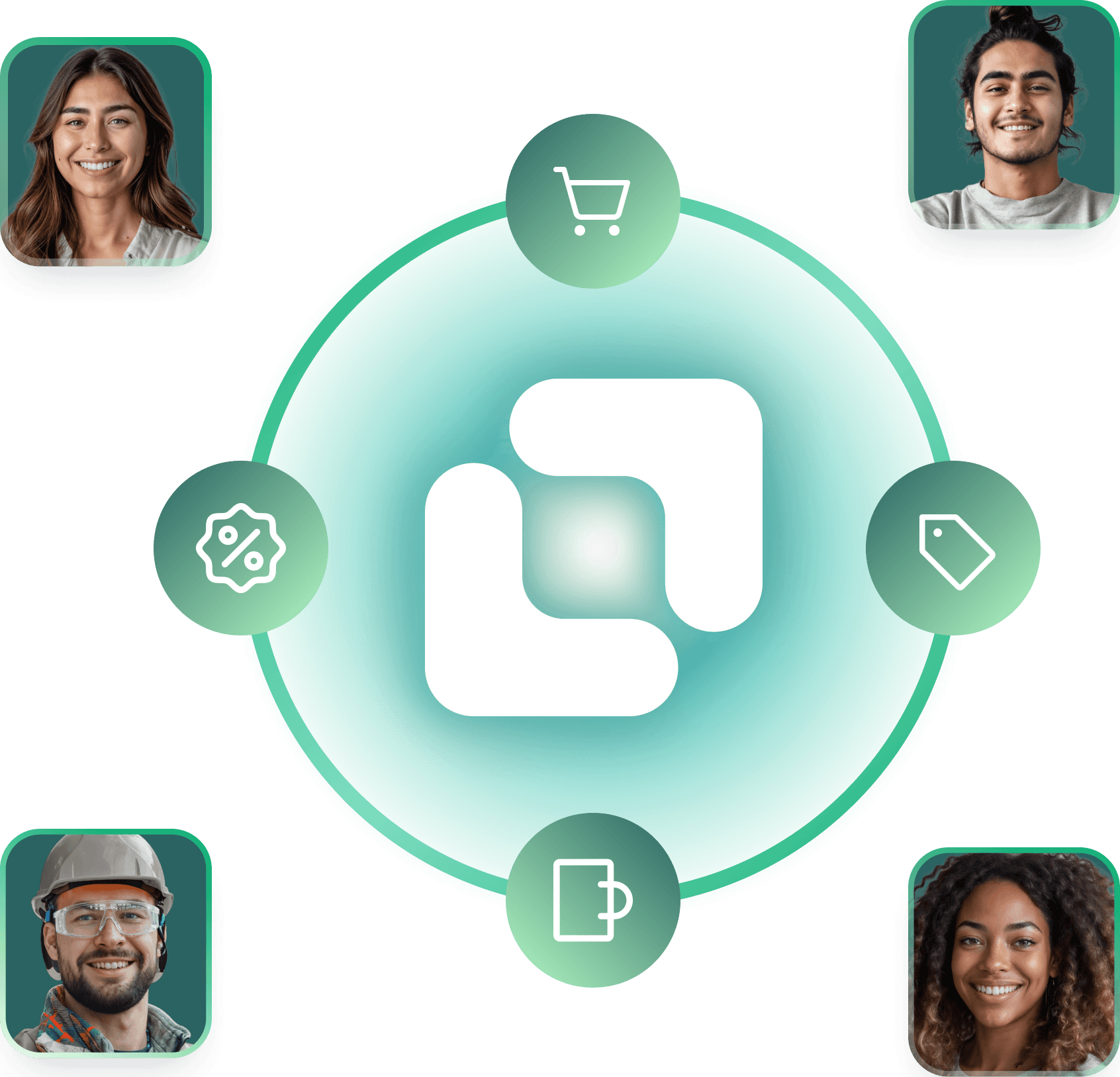
Birdseye organizes your transactional data for clarity
Birdseye analyzes purchase behavior to identify each customer's favorite menu items and offers.
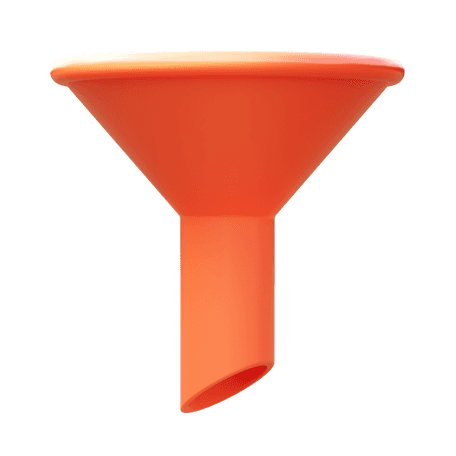
Customers receive personalized offers tailored to their preferences, boosting loyalty and satisfaction.
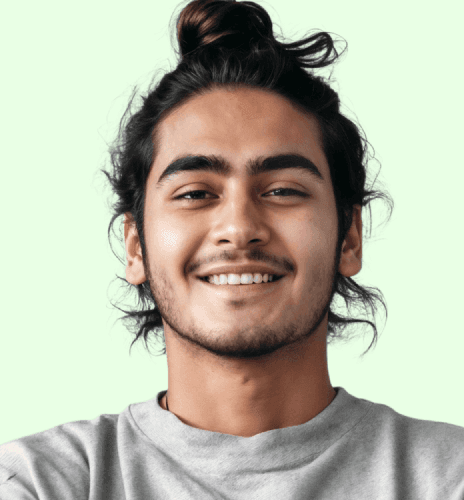
Preferences
Spicy
Takeout
Discount Sensitivity
Low
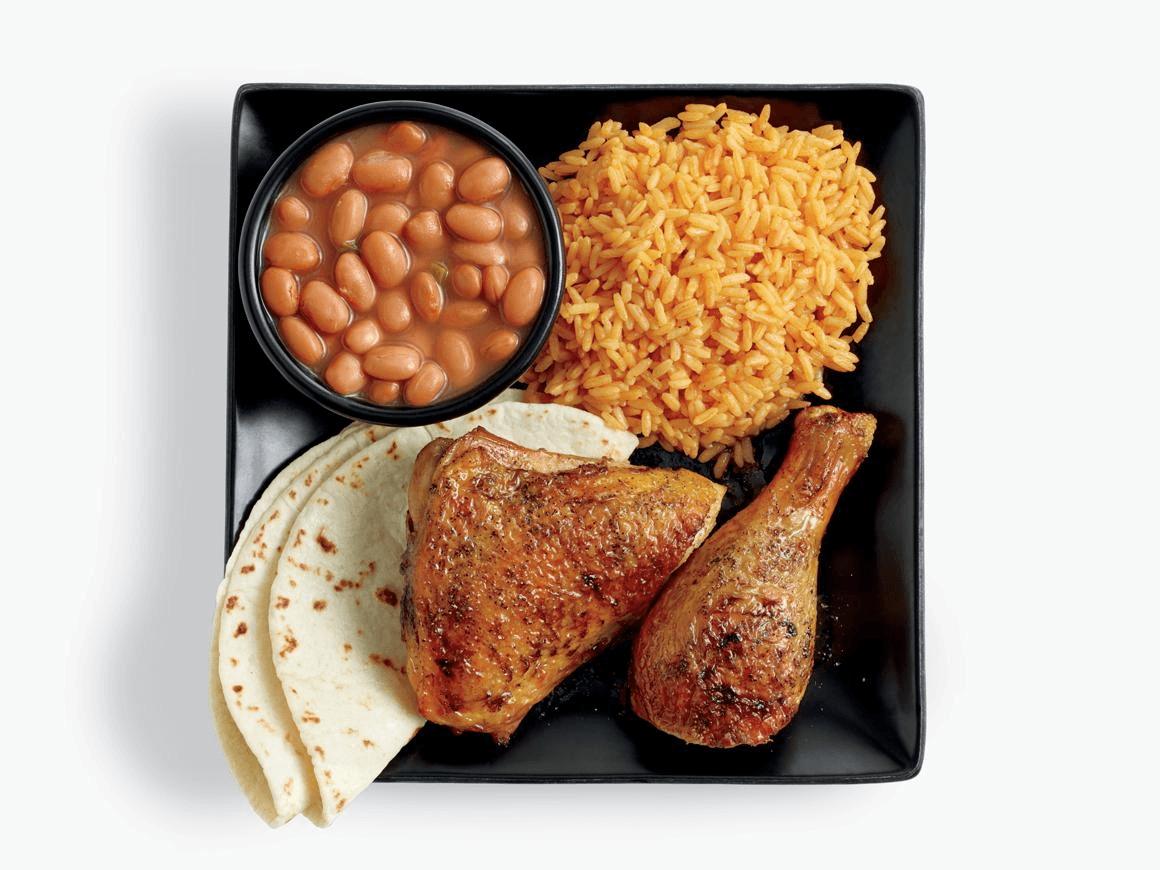
Craving Something Tasty?
Hey Diego,
Get 10% Off Your Takeout Order! Enjoy our
fire-grilled chicken with your limited time offer.
SPI_Diego342
Order now
Integrate AI-driven personalized offers across touch points:
Mobile App
Web Interface
POS System
Kiosk
Direct Mail
SMS
Custom API
Real results.
Don’t take our word for it.
Case Study
Propensity Modeling for Loblaws’ PC Optimum Generated $12BN Annually
Client Overview:
Loblaws, one of Canada’s largest grocery retailers, operates over 2,400 locations and reported $54 billion in revenue in 2023. Its loyalty program, PC Optimum, is key to this success, contributing $12-15 billion annually, with over 6 million weekly active users.
The Challenge:
Loblaws aimed to enhance customer engagement and lifetime value by personalizing offers to individual preferences and behaviors. The goal was to increase customer loyalty, visit frequency, and overall revenue per customer.
The Solution
Proprietary propensity modelling was deployed to analyze customer data, predicting individual responses to specific promotions. By creating customer clusters, Loblaws targeted high- propensity users with personalized offers through the PC Optimum app, leading to more relevant promotions, higher redemption rates, and larger basket sizes.
Key Results:
15%
Increase in Basket Sizes
High-propensity segments
saw a significant rise in transaction value & frequency.50%
Rise in Offer Redemption
Machine learning-driven offers led to better engagement versus the control group.
40%
Reduced Churn
Customers targeted with personalized offers were less likely to churn.
14%
Increase in CLV
Personalized offers boosted lifetime value compared to the control group.
Case Study
12% Revenue Lift for LCBO From Stock Optimization & Propensity Modelling
Client Overview:
The LCBO operates over 660 liquor retail locations in Ontario, serving diverse customer bases with annual revenue exceeding $6 billion. Each store caters to different demographics, influencing product demand based on factors like age, ethnicity, and income level.
The Challenge:
LCBO’s uniform product stocking approach limited its ability to meet local preferences. Stores in close proximity often had vastly different customer demands due to varying demographics, resulting in mismatches between inventory and customer needs.
The Solution
The team implemented propensity modeling to analyze customer transaction data and local demographics, providing tailored product recommendations for each store. The model considered factors like ethnicity, age, and purchase history to predict which products would perform best in specific neighborhoods. The recommendations also adjusted for seasonal and evolving trends.
Key Results:
12%
Sales Uplift
in high-density urban stores due to better product alignment.
Inventory Efficiency
through reduced stockouts and excess inventory.
Increased Customer Satisfaction
from improved inventory matches with local preferences.
Case Study
10% Revenue Lift From Optimal Pricing Platform for BG Fuels & Mobil
Client Overview:
BG Fuels, in partnership with Mobil, operates fuel stations across Canada and sought to optimize fuel pricing in a highly competitive market. Initially, they believed fuel prices were highly elastic and feared raising prices would drive away customers.
The Challenge:
BG Fuels initially believed fuel demand was highly elastic, but it proved to be inelastic. They also lacked the data and automated pricing methods to adjust their strategy, and found that local competition had less impact on market competitiveness than expected.
The Solution
Implemented a real-time pricing API that dynamically adjusted prices based on competitor rates, customer elasticity, and local demographics. This included:
Data Pipelines: Real-time competitor fuel prices were integrated into the pricing model
Competitor Matching: Significant correlation was found between local competitor prices and fuel sales, allowing for competitive pricing strategies
Demographic Targeting: Customized pricing based on local income and purchasing behavior
Real-Time API: The internal API provided optimal fuel pricing based on current market conditions and competitor data.
Key Results:
10%
Sales Uplift
through real-time pricing strategies.Improved Market Competitiveness
through competitive pricing and profit maximization in less price-sensitive scenarios
Case Study
Propensity Modeling for Loblaws’ PC Optimum Generated $12BN Annually
Client Overview:
Loblaws, one of Canada’s largest grocery retailers, operates over 2,400 locations and reported $54 billion in revenue in 2023. Its loyalty program, PC Optimum, is key to this success, contributing $12-15 billion annually, with over 6 million weekly active users.
The Challenge:
Loblaws aimed to enhance customer engagement and lifetime value by personalizing offers to individual preferences and behaviors. The goal was to increase customer loyalty, visit frequency, and overall revenue per customer.
The Solution
Proprietary propensity modelling was deployed to analyze customer data, predicting individual responses to specific promotions. By creating customer clusters, Loblaws targeted high- propensity users with personalized offers through the PC Optimum app, leading to more relevant promotions, higher redemption rates, and larger basket sizes.
Key Results:
15%
Increase in Basket Sizes
High-propensity segments
saw a significant rise in transaction value & frequency.50%
Rise in Offer Redemption
Machine learning-driven offers led to better engagement versus the control group.
40%
Reduced Churn
Customers targeted with personalized offers were less likely to churn.
14%
Increase in CLV
Personalized offers boosted lifetime value compared to the control group.
Case Study
12% Revenue Lift for LCBO From Stock Optimization & Propensity Modelling
Client Overview:
The LCBO operates over 660 liquor retail locations in Ontario, serving diverse customer bases with annual revenue exceeding $6 billion. Each store caters to different demographics, influencing product demand based on factors like age, ethnicity, and income level.
The Challenge:
LCBO’s uniform product stocking approach limited its ability to meet local preferences. Stores in close proximity often had vastly different customer demands due to varying demographics, resulting in mismatches between inventory and customer needs.
The Solution
The team implemented propensity modeling to analyze customer transaction data and local demographics, providing tailored product recommendations for each store. The model considered factors like ethnicity, age, and purchase history to predict which products would perform best in specific neighborhoods. The recommendations also adjusted for seasonal and evolving trends.
Key Results:
12%
Sales Uplift
in high-density urban stores due to better product alignment.
Inventory Efficiency
through reduced stockouts and excess inventory.
Increased Customer Satisfaction
from improved inventory matches with local preferences.
Case Study
10% Revenue Lift From Optimal Pricing Platform for BG Fuels & Mobil
Client Overview:
BG Fuels, in partnership with Mobil, operates fuel stations across Canada and sought to optimize fuel pricing in a highly competitive market. Initially, they believed fuel prices were highly elastic and feared raising prices would drive away customers.
The Challenge:
BG Fuels initially believed fuel demand was highly elastic, but it proved to be inelastic. They also lacked the data and automated pricing methods to adjust their strategy, and found that local competition had less impact on market competitiveness than expected.
The Solution
Implemented a real-time pricing API that dynamically adjusted prices based on competitor rates, customer elasticity, and local demographics. This included:
Data Pipelines: Real-time competitor fuel prices were integrated into the pricing model
Competitor Matching: Significant correlation was found between local competitor prices and fuel sales, allowing for competitive pricing strategies
Demographic Targeting: Customized pricing based on local income and purchasing behavior
Real-Time API: The internal API provided optimal fuel pricing based on current market conditions and competitor data.
Key Results:
10%
Sales Uplift
through real-time pricing strategies.Improved Market Competitiveness
through competitive pricing and profit maximization in less price-sensitive scenarios
Case Study
Propensity Modeling for Loblaws’ PC Optimum Generated $12BN Annually
Client Overview:
Loblaws, one of Canada’s largest grocery retailers, operates over 2,400 locations and reported $54 billion in revenue in 2023. Its loyalty program, PC Optimum, is key to this success, contributing $12-15 billion annually, with over 6 million weekly active users.
The Challenge:
Loblaws aimed to enhance customer engagement and lifetime value by personalizing offers to individual preferences and behaviors. The goal was to increase customer loyalty, visit frequency, and overall revenue per customer.
The Solution
Proprietary propensity modelling was deployed to analyze customer data, predicting individual responses to specific promotions. By creating customer clusters, Loblaws targeted high- propensity users with personalized offers through the PC Optimum app, leading to more relevant promotions, higher redemption rates, and larger basket sizes.
Key Results:
15%
Increase in Basket Sizes
High-propensity segments
saw a significant rise in transaction value & frequency.50%
Rise in Offer Redemption
Machine learning-driven offers led to better engagement versus the control group.
40%
Reduced Churn
Customers targeted with personalized offers were less likely to churn.
14%
Increase in CLV
Personalized offers boosted lifetime value compared to the control group.
Case Study
12% Revenue Lift for LCBO From Stock Optimization & Propensity Modelling
Client Overview:
The LCBO operates over 660 liquor retail locations in Ontario, serving diverse customer bases with annual revenue exceeding $6 billion. Each store caters to different demographics, influencing product demand based on factors like age, ethnicity, and income level.
The Challenge:
LCBO’s uniform product stocking approach limited its ability to meet local preferences. Stores in close proximity often had vastly different customer demands due to varying demographics, resulting in mismatches between inventory and customer needs.
The Solution
The team implemented propensity modeling to analyze customer transaction data and local demographics, providing tailored product recommendations for each store. The model considered factors like ethnicity, age, and purchase history to predict which products would perform best in specific neighborhoods. The recommendations also adjusted for seasonal and evolving trends.
Key Results:
12%
Sales Uplift
in high-density urban stores due to better product alignment.
Inventory Efficiency
through reduced stockouts and excess inventory.
Increased Customer Satisfaction
from improved inventory matches with local preferences.
Case Study
10% Revenue Lift From Optimal Pricing Platform for BG Fuels & Mobil
Client Overview:
BG Fuels, in partnership with Mobil, operates fuel stations across Canada and sought to optimize fuel pricing in a highly competitive market. Initially, they believed fuel prices were highly elastic and feared raising prices would drive away customers.
The Challenge:
BG Fuels initially believed fuel demand was highly elastic, but it proved to be inelastic. They also lacked the data and automated pricing methods to adjust their strategy, and found that local competition had less impact on market competitiveness than expected.
The Solution
Implemented a real-time pricing API that dynamically adjusted prices based on competitor rates, customer elasticity, and local demographics. This included:
Data Pipelines: Real-time competitor fuel prices were integrated into the pricing model
Competitor Matching: Significant correlation was found between local competitor prices and fuel sales, allowing for competitive pricing strategies
Demographic Targeting: Customized pricing based on local income and purchasing behavior
Real-Time API: The internal API provided optimal fuel pricing based on current market conditions and competitor data.
Key Results:
10%
Sales Uplift
through real-time pricing strategies.Improved Market Competitiveness
through competitive pricing and profit maximization in less price-sensitive scenarios
Case Study
Propensity Modeling for Loblaws’ PC Optimum Generated $12BN Annually
Client Overview:
Loblaws, one of Canada’s largest grocery retailers, operates over 2,400 locations and reported $54 billion in revenue in 2023. Its loyalty program, PC Optimum, is key to this success, contributing $12-15 billion annually, with over 6 million weekly active users.
The Challenge:
Loblaws aimed to enhance customer engagement and lifetime value by personalizing offers to individual preferences and behaviors. The goal was to increase customer loyalty, visit frequency, and overall revenue per customer.
The Solution
Proprietary propensity modelling was deployed to analyze customer data, predicting individual responses to specific promotions. By creating customer clusters, Loblaws targeted high- propensity users with personalized offers through the PC Optimum app, leading to more relevant promotions, higher redemption rates, and larger basket sizes.
Key Results:
15%
Increase in Basket Sizes
High-propensity segments
saw a significant rise in transaction value & frequency.50%
Rise in Offer Redemption
Machine learning-driven offers led to better engagement versus the control group.
40%
Reduced Churn
Customers targeted with personalized offers were less likely to churn.
14%
Increase in CLV
Personalized offers boosted lifetime value compared to the control group.
Case Study
12% Revenue Lift for LCBO From Stock Optimization & Propensity Modelling
Client Overview:
The LCBO operates over 660 liquor retail locations in Ontario, serving diverse customer bases with annual revenue exceeding $6 billion. Each store caters to different demographics, influencing product demand based on factors like age, ethnicity, and income level.
The Challenge:
LCBO’s uniform product stocking approach limited its ability to meet local preferences. Stores in close proximity often had vastly different customer demands due to varying demographics, resulting in mismatches between inventory and customer needs.
The Solution
The team implemented propensity modeling to analyze customer transaction data and local demographics, providing tailored product recommendations for each store. The model considered factors like ethnicity, age, and purchase history to predict which products would perform best in specific neighborhoods. The recommendations also adjusted for seasonal and evolving trends.
Key Results:
12%
Sales Uplift
in high-density urban stores due to better product alignment.
Inventory Efficiency
through reduced stockouts and excess inventory.
Increased Customer Satisfaction
from improved inventory matches with local preferences.
Case Study
10% Revenue Lift From Optimal Pricing Platform for BG Fuels & Mobil
Client Overview:
BG Fuels, in partnership with Mobil, operates fuel stations across Canada and sought to optimize fuel pricing in a highly competitive market. Initially, they believed fuel prices were highly elastic and feared raising prices would drive away customers.
The Challenge:
BG Fuels initially believed fuel demand was highly elastic, but it proved to be inelastic. They also lacked the data and automated pricing methods to adjust their strategy, and found that local competition had less impact on market competitiveness than expected.
The Solution
Implemented a real-time pricing API that dynamically adjusted prices based on competitor rates, customer elasticity, and local demographics. This included:
Data Pipelines: Real-time competitor fuel prices were integrated into the pricing model
Competitor Matching: Significant correlation was found between local competitor prices and fuel sales, allowing for competitive pricing strategies
Demographic Targeting: Customized pricing based on local income and purchasing behavior
Real-Time API: The internal API provided optimal fuel pricing based on current market conditions and competitor data.
Key Results:
10%
Sales Uplift
through real-time pricing strategies.Improved Market Competitiveness
through competitive pricing and profit maximization in less price-sensitive scenarios
Case Study
Propensity Modeling for Loblaws’ PC Optimum Generated $12BN Annually
Client Overview:
Loblaws, one of Canada’s largest grocery retailers, operates over 2,400 locations and reported $54 billion in revenue in 2023. Its loyalty program, PC Optimum, is key to this success, contributing $12-15 billion annually, with over 6 million weekly active users.
The Challenge:
Loblaws aimed to enhance customer engagement and lifetime value by personalizing offers to individual preferences and behaviors. The goal was to increase customer loyalty, visit frequency, and overall revenue per customer.
The Solution
Proprietary propensity modelling was deployed to analyze customer data, predicting individual responses to specific promotions. By creating customer clusters, Loblaws targeted high- propensity users with personalized offers through the PC Optimum app, leading to more relevant promotions, higher redemption rates, and larger basket sizes.
Key Results:
15%
Increase in Basket Sizes
High-propensity segments
saw a significant rise in transaction value & frequency.50%
Rise in Offer Redemption
Machine learning-driven offers led to better engagement versus the control group.
40%
Reduced Churn
Customers targeted with personalized offers were less likely to churn.
14%
Increase in CLV
Personalized offers boosted lifetime value compared to the control group.
Case Study
Propensity Modeling for Loblaws’ PC Optimum Generated $12BN Annually
LCBO’s uniform product stocking approach limited its ability to meet local preferences. Stores in close proximity often had vastly different customer demands due to varying demographics, resulting in mismatches between inventory and customer needs.
Client Overview:
The LCBO operates over 660 liquor retail locations in Ontario, serving diverse customer bases with annual revenue exceeding $6 billion. Each store caters to different demographics, influencing product demand based on factors like age, ethnicity, and income level.
The Solution
The team implemented propensity modeling to analyze customer transaction data and local demographics, providing tailored product recommendations for each store. The model considered factors like ethnicity, age, and purchase history to predict which products would perform best in specific neighborhoods. The recommendations also adjusted for seasonal and evolving trends.
Key Results:
12%
Sales Uplift
in high-density urban stores due to better product alignment.Increased Customer Satisfaction
from improved inventory matches with local preferences.
Inventory Efficiency
through reduced stockouts and excess inventory.
Case Study
10% Revenue Lift From Optimal Pricing Platform for BG Fuels & Mobil
Client Overview:
BG Fuels, in partnership with Mobil, operates fuel stations across Canada and sought to optimize fuel pricing in a highly competitive market. Initially, they believed fuel prices were highly elastic and feared raising prices would drive away customers.
The Challenge:
BG Fuels initially believed fuel demand was highly elastic, but it proved to be inelastic. They also lacked the data and automated pricing methods to adjust their strategy, and found that local competition had less impact on market competitiveness than expected.
The Solution
Implemented a real-time pricing API that dynamically adjusted prices based on competitor rates, customer elasticity, and local demographics. This included:
Data Pipelines: Real-time competitor fuel prices were integrated into the pricing model
Competitor Matching: Significant correlation was found between local competitor prices and fuel sales, allowing for competitive pricing strategies
Demographic Targeting: Customized pricing based on local income and purchasing behavior
Real-Time API: The internal API provided optimal fuel pricing based on current market conditions and competitor data.
Key Results:
10%
Sales Uplift
through real-time pricing strategies.
Improved Market Competitiveness
through competitive pricing and profit maximization in less price-sensitive scenarios
Increased Customer Loyalty
through dynamic pricing aligned with local demographic needs.
Case Study
Propensity Modeling for Loblaws’ PC Optimum Generated $12BN Annually
Client Overview:
Loblaws, one of Canada’s largest grocery retailers, operates over 2,400 locations and reported $54 billion in revenue in 2023. Its loyalty program, PC Optimum, is key to this success, contributing $12-15 billion annually, with over 6 million weekly active users.
The Challenge:
Loblaws aimed to enhance customer engagement and lifetime value by personalizing offers to individual preferences and behaviors. The goal was to increase customer loyalty, visit frequency, and overall revenue per customer.
The Solution
Proprietary propensity modelling was deployed to analyze customer data, predicting individual responses to specific promotions. By creating customer clusters, Loblaws targeted high- propensity users with personalized offers through the PC Optimum app, leading to more relevant promotions, higher redemption rates, and larger basket sizes.
Key Results:
15%
Increase in Basket Sizes
High-propensity segments
saw a significant rise in transaction value & frequency.50%
Rise in Offer Redemption
Machine learning-driven offers led to better engagement versus the control group.
40%
Reduced Churn
Customers targeted with personalized offers were less likely to churn.
14%
Increase in CLV
Personalized offers boosted lifetime value compared to the control group.
Case Study
Propensity Modeling for Loblaws’ PC Optimum Generated $12BN Annually
LCBO’s uniform product stocking approach limited its ability to meet local preferences. Stores in close proximity often had vastly different customer demands due to varying demographics, resulting in mismatches between inventory and customer needs.
Client Overview:
The LCBO operates over 660 liquor retail locations in Ontario, serving diverse customer bases with annual revenue exceeding $6 billion. Each store caters to different demographics, influencing product demand based on factors like age, ethnicity, and income level.
The Solution
The team implemented propensity modeling to analyze customer transaction data and local demographics, providing tailored product recommendations for each store. The model considered factors like ethnicity, age, and purchase history to predict which products would perform best in specific neighborhoods. The recommendations also adjusted for seasonal and evolving trends.
Key Results:
12%
Sales Uplift
in high-density urban stores due to better product alignment.Increased Customer Satisfaction
from improved inventory matches with local preferences.
Inventory Efficiency
through reduced stockouts and excess inventory.
Case Study
10% Revenue Lift From Optimal Pricing Platform for BG Fuels & Mobil
Client Overview:
BG Fuels, in partnership with Mobil, operates fuel stations across Canada and sought to optimize fuel pricing in a highly competitive market. Initially, they believed fuel prices were highly elastic and feared raising prices would drive away customers.
The Challenge:
BG Fuels initially believed fuel demand was highly elastic, but it proved to be inelastic. They also lacked the data and automated pricing methods to adjust their strategy, and found that local competition had less impact on market competitiveness than expected.
The Solution
Implemented a real-time pricing API that dynamically adjusted prices based on competitor rates, customer elasticity, and local demographics. This included:
Data Pipelines: Real-time competitor fuel prices were integrated into the pricing model
Competitor Matching: Significant correlation was found between local competitor prices and fuel sales, allowing for competitive pricing strategies
Demographic Targeting: Customized pricing based on local income and purchasing behavior
Real-Time API: The internal API provided optimal fuel pricing based on current market conditions and competitor data.
Key Results:
10%
Sales Uplift
through real-time pricing strategies.
Improved Market Competitiveness
through competitive pricing and profit maximization in less price-sensitive scenarios
Increased Customer Loyalty
through dynamic pricing aligned with local demographic needs.
Case Study
Propensity Modeling for Loblaws’ PC Optimum Generated $12BN Annually
Client Overview:
Loblaws, one of Canada’s largest grocery retailers, operates over 2,400 locations and reported $54 billion in revenue in 2023. Its loyalty program, PC Optimum, is key to this success, contributing $12-15 billion annually, with over 6 million weekly active users.
The Challenge:
Loblaws aimed to enhance customer engagement and lifetime value by personalizing offers to individual preferences and behaviors. The goal was to increase customer loyalty, visit frequency, and overall revenue per customer.
The Solution
Proprietary propensity modelling was deployed to analyze customer data, predicting individual responses to specific promotions. By creating customer clusters, Loblaws targeted high- propensity users with personalized offers through the PC Optimum app, leading to more relevant promotions, higher redemption rates, and larger basket sizes.
Key Results:
15%
Increase in Basket Sizes
High-propensity segments
saw a significant rise in transaction value & frequency.50%
Rise in Offer Redemption
Machine learning-driven offers led to better engagement versus the control group.
40%
Reduced Churn
Customers targeted with personalized offers were less likely to churn.
14%
Increase in CLV
Personalized offers boosted lifetime value compared to the control group.
Case Study
Propensity Modeling for Loblaws’ PC Optimum Generated $12BN Annually
LCBO’s uniform product stocking approach limited its ability to meet local preferences. Stores in close proximity often had vastly different customer demands due to varying demographics, resulting in mismatches between inventory and customer needs.
Client Overview:
The LCBO operates over 660 liquor retail locations in Ontario, serving diverse customer bases with annual revenue exceeding $6 billion. Each store caters to different demographics, influencing product demand based on factors like age, ethnicity, and income level.
The Solution
The team implemented propensity modeling to analyze customer transaction data and local demographics, providing tailored product recommendations for each store. The model considered factors like ethnicity, age, and purchase history to predict which products would perform best in specific neighborhoods. The recommendations also adjusted for seasonal and evolving trends.
Key Results:
12%
Sales Uplift
in high-density urban stores due to better product alignment.Increased Customer Satisfaction
from improved inventory matches with local preferences.
Inventory Efficiency
through reduced stockouts and excess inventory.
Case Study
10% Revenue Lift From Optimal Pricing Platform for BG Fuels & Mobil
Client Overview:
BG Fuels, in partnership with Mobil, operates fuel stations across Canada and sought to optimize fuel pricing in a highly competitive market. Initially, they believed fuel prices were highly elastic and feared raising prices would drive away customers.
The Challenge:
BG Fuels initially believed fuel demand was highly elastic, but it proved to be inelastic. They also lacked the data and automated pricing methods to adjust their strategy, and found that local competition had less impact on market competitiveness than expected.
The Solution
Implemented a real-time pricing API that dynamically adjusted prices based on competitor rates, customer elasticity, and local demographics. This included:
Data Pipelines: Real-time competitor fuel prices were integrated into the pricing model
Competitor Matching: Significant correlation was found between local competitor prices and fuel sales, allowing for competitive pricing strategies
Demographic Targeting: Customized pricing based on local income and purchasing behavior
Real-Time API: The internal API provided optimal fuel pricing based on current market conditions and competitor data.
Key Results:
10%
Sales Uplift
through real-time pricing strategies.
Improved Market Competitiveness
through competitive pricing and profit maximization in less price-sensitive scenarios
Increased Customer Loyalty
through dynamic pricing aligned with local demographic needs.
Case Study
Propensity Modeling for Loblaws’ PC Optimum Generated $12BN Annually
Client Overview:
Loblaws, one of Canada’s largest grocery retailers, operates over 2,400 locations and reported $54 billion in revenue in 2023. Its loyalty program, PC Optimum, is key to this success, contributing $12-15 billion annually, with over 6 million weekly active users.
The Challenge:
Loblaws aimed to enhance customer engagement and lifetime value by personalizing offers to individual preferences and behaviors. The goal was to increase customer loyalty, visit frequency, and overall revenue per customer.
The Solution
Proprietary propensity modelling was deployed to analyze customer data, predicting individual responses to specific promotions. By creating customer clusters, Loblaws targeted high- propensity users with personalized offers through the PC Optimum app, leading to more relevant promotions, higher redemption rates, and larger basket sizes.
Key Results:
15%
Increase in Basket Sizes
High-propensity segments
saw a significant rise in transaction value & frequency.50%
Rise in Offer Redemption
Machine learning-driven offers led to better engagement versus the control group.
40%
Reduced Churn
Customers targeted with personalized offers were less likely to churn.
14%
Increase in CLV
Personalized offers boosted lifetime value compared to the control group.
Case Study
Propensity Modeling for Loblaws’ PC Optimum Generated $12BN Annually
LCBO’s uniform product stocking approach limited its ability to meet local preferences. Stores in close proximity often had vastly different customer demands due to varying demographics, resulting in mismatches between inventory and customer needs.
Client Overview:
The LCBO operates over 660 liquor retail locations in Ontario, serving diverse customer bases with annual revenue exceeding $6 billion. Each store caters to different demographics, influencing product demand based on factors like age, ethnicity, and income level.
The Solution
The team implemented propensity modeling to analyze customer transaction data and local demographics, providing tailored product recommendations for each store. The model considered factors like ethnicity, age, and purchase history to predict which products would perform best in specific neighborhoods. The recommendations also adjusted for seasonal and evolving trends.
Key Results:
12%
Sales Uplift
in high-density urban stores due to better product alignment.Increased Customer Satisfaction
from improved inventory matches with local preferences.
Inventory Efficiency
through reduced stockouts and excess inventory.
Case Study
10% Revenue Lift From Optimal Pricing Platform for BG Fuels & Mobil
Client Overview:
BG Fuels, in partnership with Mobil, operates fuel stations across Canada and sought to optimize fuel pricing in a highly competitive market. Initially, they believed fuel prices were highly elastic and feared raising prices would drive away customers.
The Challenge:
BG Fuels initially believed fuel demand was highly elastic, but it proved to be inelastic. They also lacked the data and automated pricing methods to adjust their strategy, and found that local competition had less impact on market competitiveness than expected.
The Solution
Implemented a real-time pricing API that dynamically adjusted prices based on competitor rates, customer elasticity, and local demographics. This included:
Data Pipelines: Real-time competitor fuel prices were integrated into the pricing model
Competitor Matching: Significant correlation was found between local competitor prices and fuel sales, allowing for competitive pricing strategies
Demographic Targeting: Customized pricing based on local income and purchasing behavior
Real-Time API: The internal API provided optimal fuel pricing based on current market conditions and competitor data.
Key Results:
10%
Sales Uplift
through real-time pricing strategies.
Improved Market Competitiveness
through competitive pricing and profit maximization in less price-sensitive scenarios
Increased Customer Loyalty
through dynamic pricing aligned with local demographic needs.
Case Study
Propensity Modeling for Loblaws’ PC Optimum Generated $12BN Annually
Client Overview:
Loblaws, one of Canada’s largest grocery retailers, operates over 2,400 locations and reported $54 billion in revenue in 2023. Its loyalty program, PC Optimum, is key to this success, contributing $12-15 billion annually, with over 6 million weekly active users.
The Challenge:
Loblaws aimed to enhance customer engagement and lifetime value by personalizing offers to individual preferences and behaviors. The goal was to increase customer loyalty, visit frequency, and overall revenue per customer.
The Solution
Proprietary propensity modelling was deployed to analyze customer data, predicting individual responses to specific promotions. By creating customer clusters, Loblaws targeted high- propensity users with personalized offers through the PC Optimum app, leading to more relevant promotions, higher redemption rates, and larger basket sizes.
Key Results:
15%
Increase in Basket Sizes
High-propensity segments
saw a significant rise in transaction value & frequency.50%
Rise in Offer Redemption
Machine learning-driven offers led to better engagement versus the control group.
40%
Reduced Churn
Customers targeted with personalized offers were less likely to churn.
14%
Increase in CLV
Personalized offers boosted lifetime value compared to the control group.
Case Study
Propensity Modeling for Loblaws’ PC Optimum Generated $12BN Annually
LCBO’s uniform product stocking approach limited its ability to meet local preferences. Stores in close proximity often had vastly different customer demands due to varying demographics, resulting in mismatches between inventory and customer needs.
Client Overview:
The LCBO operates over 660 liquor retail locations in Ontario, serving diverse customer bases with annual revenue exceeding $6 billion. Each store caters to different demographics, influencing product demand based on factors like age, ethnicity, and income level.
The Solution
The team implemented propensity modeling to analyze customer transaction data and local demographics, providing tailored product recommendations for each store. The model considered factors like ethnicity, age, and purchase history to predict which products would perform best in specific neighborhoods. The recommendations also adjusted for seasonal and evolving trends.
Key Results:
12%
Sales Uplift
in high-density urban stores due to better product alignment.Increased Customer Satisfaction
from improved inventory matches with local preferences.
Inventory Efficiency
through reduced stockouts and excess inventory.
Case Study
10% Revenue Lift From Optimal Pricing Platform for BG Fuels & Mobil
Client Overview:
BG Fuels, in partnership with Mobil, operates fuel stations across Canada and sought to optimize fuel pricing in a highly competitive market. Initially, they believed fuel prices were highly elastic and feared raising prices would drive away customers.
The Challenge:
BG Fuels initially believed fuel demand was highly elastic, but it proved to be inelastic. They also lacked the data and automated pricing methods to adjust their strategy, and found that local competition had less impact on market competitiveness than expected.
The Solution
Implemented a real-time pricing API that dynamically adjusted prices based on competitor rates, customer elasticity, and local demographics. This included:
Data Pipelines: Real-time competitor fuel prices were integrated into the pricing model
Competitor Matching: Significant correlation was found between local competitor prices and fuel sales, allowing for competitive pricing strategies
Demographic Targeting: Customized pricing based on local income and purchasing behavior
Real-Time API: The internal API provided optimal fuel pricing based on current market conditions and competitor data.
Key Results:
10%
Sales Uplift
through real-time pricing strategies.
Improved Market Competitiveness
through competitive pricing and profit maximization in less price-sensitive scenarios
Increased Customer Loyalty
through dynamic pricing aligned with local demographic needs.
Case Study
Propensity Modeling for Loblaws’ PC Optimum Generated $12BN Annually
Client Overview:
Loblaws, one of Canada’s largest grocery retailers, operates over 2,400 locations and reported $54 billion in revenue in 2023. Its loyalty program, PC Optimum, is key to this success, contributing $12-15 billion annually, with over 6 million weekly active users.
The Challenge:
Loblaws aimed to enhance customer engagement and lifetime value by personalizing offers to individual preferences and behaviors. The goal was to increase customer loyalty, visit frequency, and overall revenue per customer.
The Solution
Proprietary propensity modelling was deployed to analyze customer data, predicting individual responses to specific promotions. By creating customer clusters, Loblaws targeted high- propensity users with personalized offers through the PC Optimum app, leading to more relevant promotions, higher redemption rates, and larger basket sizes.
Key Results:
15%
Increase in Basket Sizes
High-propensity segments
saw a significant rise in transaction value & frequency.50%
Rise in Offer Redemption
Machine learning-driven offers led to better engagement versus the control group.
40%
Reduced Churn
Customers targeted with personalized offers were less likely to churn.
14%
Increase in CLV
Personalized offers boosted lifetime value compared to the control group.
Case Study
Propensity Modeling for Loblaws’ PC Optimum Generated $12BN Annually
LCBO’s uniform product stocking approach limited its ability to meet local preferences. Stores in close proximity often had vastly different customer demands due to varying demographics, resulting in mismatches between inventory and customer needs.
Client Overview:
The LCBO operates over 660 liquor retail locations in Ontario, serving diverse customer bases with annual revenue exceeding $6 billion. Each store caters to different demographics, influencing product demand based on factors like age, ethnicity, and income level.
The Solution
The team implemented propensity modeling to analyze customer transaction data and local demographics, providing tailored product recommendations for each store. The model considered factors like ethnicity, age, and purchase history to predict which products would perform best in specific neighborhoods. The recommendations also adjusted for seasonal and evolving trends.
Key Results:
12%
Sales Uplift
in high-density urban stores due to better product alignment.Increased Customer Satisfaction
from improved inventory matches with local preferences.
Inventory Efficiency
through reduced stockouts and excess inventory.
Case Study
10% Revenue Lift From Optimal Pricing Platform for BG Fuels & Mobil
Client Overview:
BG Fuels, in partnership with Mobil, operates fuel stations across Canada and sought to optimize fuel pricing in a highly competitive market. Initially, they believed fuel prices were highly elastic and feared raising prices would drive away customers.
The Challenge:
BG Fuels initially believed fuel demand was highly elastic, but it proved to be inelastic. They also lacked the data and automated pricing methods to adjust their strategy, and found that local competition had less impact on market competitiveness than expected.
The Solution
Implemented a real-time pricing API that dynamically adjusted prices based on competitor rates, customer elasticity, and local demographics. This included:
Data Pipelines: Real-time competitor fuel prices were integrated into the pricing model
Competitor Matching: Significant correlation was found between local competitor prices and fuel sales, allowing for competitive pricing strategies
Demographic Targeting: Customized pricing based on local income and purchasing behavior
Real-Time API: The internal API provided optimal fuel pricing based on current market conditions and competitor data.
Key Results:
10%
Sales Uplift
through real-time pricing strategies.
Improved Market Competitiveness
through competitive pricing and profit maximization in less price-sensitive scenarios
Increased Customer Loyalty
through dynamic pricing aligned with local demographic needs.
Case Study
Propensity Modeling for Loblaws’ PC Optimum Generated $12BN Annually
Client Overview:
Loblaws, one of Canada’s largest grocery retailers, operates over 2,400 locations and reported $54 billion in revenue in 2023. Its loyalty program, PC Optimum, is key to this success, contributing $12-15 billion annually, with over 6 million weekly active users.
The Challenge:
Loblaws aimed to enhance customer engagement and lifetime value by personalizing offers to individual preferences and behaviors. The goal was to increase customer loyalty, visit frequency, and overall revenue per customer.
The Solution
Proprietary propensity modelling was deployed to analyze customer data, predicting individual responses to specific promotions. By creating customer clusters, Loblaws targeted high- propensity users with personalized offers through the PC Optimum app, leading to more relevant promotions, higher redemption rates, and larger basket sizes.
Key Results:
15%
Increase in Basket Sizes
High-propensity segments
saw a significant rise in transaction value & frequency.50%
Rise in Offer Redemption
Machine learning-driven offers led to better engagement versus the control group.
40%
Reduced Churn
Customers targeted with personalized offers were less likely to churn.
14%
Increase in CLV
Personalized offers boosted lifetime value compared to the control group.
Case Study
Propensity Modeling for Loblaws’ PC Optimum Generated $12BN Annually
LCBO’s uniform product stocking approach limited its ability to meet local preferences. Stores in close proximity often had vastly different customer demands due to varying demographics, resulting in mismatches between inventory and customer needs.
Client Overview:
The LCBO operates over 660 liquor retail locations in Ontario, serving diverse customer bases with annual revenue exceeding $6 billion. Each store caters to different demographics, influencing product demand based on factors like age, ethnicity, and income level.
The Solution
The team implemented propensity modeling to analyze customer transaction data and local demographics, providing tailored product recommendations for each store. The model considered factors like ethnicity, age, and purchase history to predict which products would perform best in specific neighborhoods. The recommendations also adjusted for seasonal and evolving trends.
Key Results:
12%
Sales Uplift
in high-density urban stores due to better product alignment.Increased Customer Satisfaction
from improved inventory matches with local preferences.
Inventory Efficiency
through reduced stockouts and excess inventory.
Case Study
10% Revenue Lift From Optimal Pricing Platform for BG Fuels & Mobil
Client Overview:
BG Fuels, in partnership with Mobil, operates fuel stations across Canada and sought to optimize fuel pricing in a highly competitive market. Initially, they believed fuel prices were highly elastic and feared raising prices would drive away customers.
The Challenge:
BG Fuels initially believed fuel demand was highly elastic, but it proved to be inelastic. They also lacked the data and automated pricing methods to adjust their strategy, and found that local competition had less impact on market competitiveness than expected.
The Solution
Implemented a real-time pricing API that dynamically adjusted prices based on competitor rates, customer elasticity, and local demographics. This included:
Data Pipelines: Real-time competitor fuel prices were integrated into the pricing model
Competitor Matching: Significant correlation was found between local competitor prices and fuel sales, allowing for competitive pricing strategies
Demographic Targeting: Customized pricing based on local income and purchasing behavior
Real-Time API: The internal API provided optimal fuel pricing based on current market conditions and competitor data.
Key Results:
10%
Sales Uplift
through real-time pricing strategies.
Improved Market Competitiveness
through competitive pricing and profit maximization in less price-sensitive scenarios
Increased Customer Loyalty
through dynamic pricing aligned with local demographic needs.
Case Study
Propensity Modeling for Loblaws’ PC Optimum Generated $12BN Annually
Client Overview:
Loblaws, one of Canada’s largest grocery retailers, operates over 2,400 locations and reported $54 billion in revenue in 2023. Its loyalty program, PC Optimum, is key to this success, contributing $12-15 billion annually, with over 6 million weekly active users.
The Challenge:
Loblaws aimed to enhance customer engagement and lifetime value by personalizing offers to individual preferences and behaviors. The goal was to increase customer loyalty, visit frequency, and overall revenue per customer.
The Solution
Proprietary propensity modelling was deployed to analyze customer data, predicting individual responses to specific promotions. By creating customer clusters, Loblaws targeted high- propensity users with personalized offers through the PC Optimum app, leading to more relevant promotions, higher redemption rates, and larger basket sizes.
Key Results:
15%
Increase in Basket Sizes
High-propensity segments
saw a significant rise in transaction value & frequency.50%
Rise in Offer Redemption
Machine learning-driven offers led to better engagement versus the control group.
40%
Reduced Churn
Customers targeted with personalized offers were less likely to churn.
14%
Increase in CLV
Personalized offers boosted lifetime value compared to the control group.
Case Study
Propensity Modeling for Loblaws’ PC Optimum Generated $12BN Annually
LCBO’s uniform product stocking approach limited its ability to meet local preferences. Stores in close proximity often had vastly different customer demands due to varying demographics, resulting in mismatches between inventory and customer needs.
Client Overview:
The LCBO operates over 660 liquor retail locations in Ontario, serving diverse customer bases with annual revenue exceeding $6 billion. Each store caters to different demographics, influencing product demand based on factors like age, ethnicity, and income level.
The Solution
The team implemented propensity modeling to analyze customer transaction data and local demographics, providing tailored product recommendations for each store. The model considered factors like ethnicity, age, and purchase history to predict which products would perform best in specific neighborhoods. The recommendations also adjusted for seasonal and evolving trends.
Key Results:
12%
Sales Uplift
in high-density urban stores due to better product alignment.Increased Customer Satisfaction
from improved inventory matches with local preferences.
Inventory Efficiency
through reduced stockouts and excess inventory.
Case Study
10% Revenue Lift From Optimal Pricing Platform for BG Fuels & Mobil
Client Overview:
BG Fuels, in partnership with Mobil, operates fuel stations across Canada and sought to optimize fuel pricing in a highly competitive market. Initially, they believed fuel prices were highly elastic and feared raising prices would drive away customers.
The Challenge:
BG Fuels initially believed fuel demand was highly elastic, but it proved to be inelastic. They also lacked the data and automated pricing methods to adjust their strategy, and found that local competition had less impact on market competitiveness than expected.
The Solution
Implemented a real-time pricing API that dynamically adjusted prices based on competitor rates, customer elasticity, and local demographics. This included:
Data Pipelines: Real-time competitor fuel prices were integrated into the pricing model
Competitor Matching: Significant correlation was found between local competitor prices and fuel sales, allowing for competitive pricing strategies
Demographic Targeting: Customized pricing based on local income and purchasing behavior
Real-Time API: The internal API provided optimal fuel pricing based on current market conditions and competitor data.
Key Results:
10%
Sales Uplift
through real-time pricing strategies.
Improved Market Competitiveness
through competitive pricing and profit maximization in less price-sensitive scenarios
Increased Customer Loyalty
through dynamic pricing aligned with local demographic needs.
About us
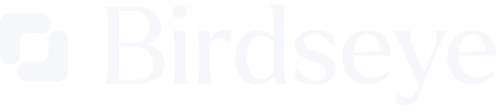
Birdseye is a venture-backed technology company specializing in shopper propensity modeling, driving increased lifetime value, customer loyalty, and brand affinity for leading retailers and businesses.
Birdseye is a venture-backed technology company specializing in shopper propensity modeling, driving increased lifetime value, customer loyalty, and brand affinity for leading retailers and businesses.
Birdseye is a venture-backed technology company specializing in shopper propensity modeling, driving increased lifetime value, customer loyalty, and brand affinity for leading retailers and businesses.
Investors and Advisors

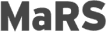
Birdseye in the news
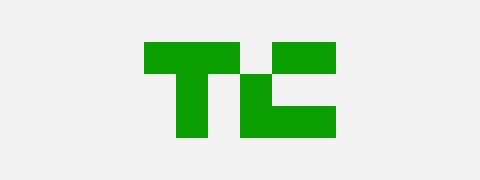
November 22, 2023
Birdseye’s AI-powered chief marketing officer drives personalized email campaigns for retailers
Birdseye’s AI-powered chief marketing officer drives personalized email campaigns for retailers
Birdseye’s AI-powered chief marketing officer drives personalized email campaigns for retailers
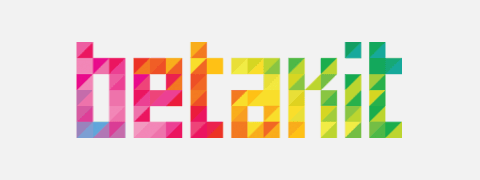
November 22, 2023
Birdseye Closes $4.1 Million CAD in Seed Funding for an AI-Powered Marketing Platform.
Birdseye Closes $4.1 Million CAD in Seed Funding for an AI-Powered Marketing Platform.
Birdseye Closes $4.1 Million CAD in Seed Funding for an AI-Powered Marketing Platform.
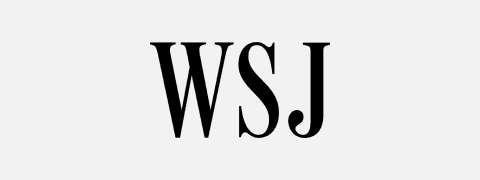
May 1, 2024
Birdseye, an AI startup in Toronto, received an initial funding of $3 million from Drive Capital.
Birdseye, an AI startup in Toronto, received an initial funding of $3 million from Drive Capital.
Birdseye, an AI startup in Toronto, received an initial funding of $3 million from Drive Capital.
Meet the Team
Experts in AI & Data Science
Birdseye's team brings over a decade of expertise in data science, machine learning and applied analytics. With a proven track record of boosting profitability through advanced propensity models, we are dedicated to unlocking the full potential of your data to drive growth and success.
Matt Bogoroch
CEO and Co-founder
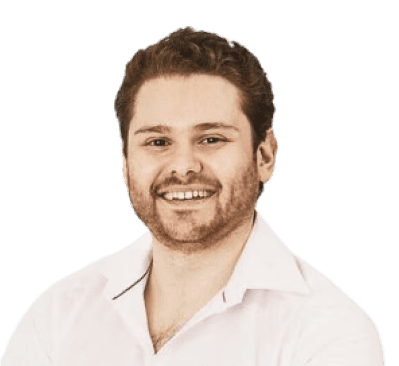
Matt Bogoroch
CEO and Co-founder
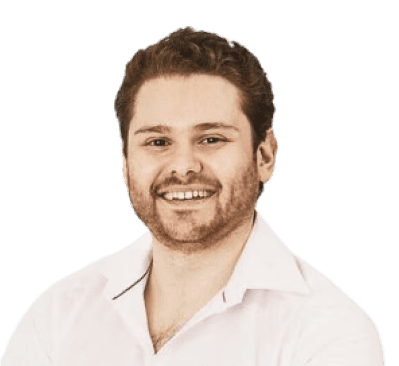
Matt Bogoroch
CEO and Co-founder
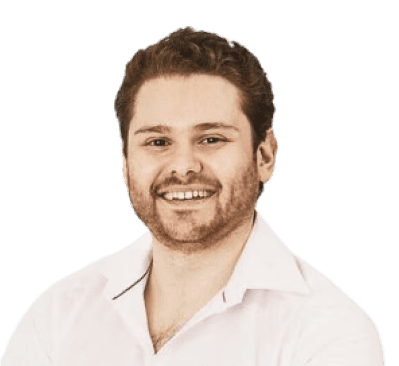
Shardul Frey
CEO and Co-founder
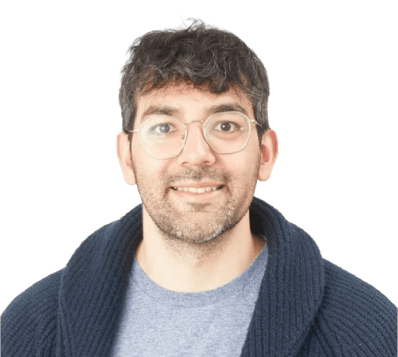
Shardul Frey
CEO and Co-founder
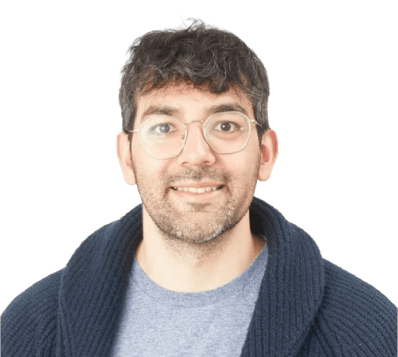
Shardul Frey
CEO and Co-founder
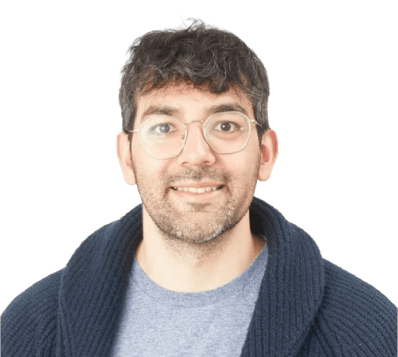
Aaron Li
Head of AI
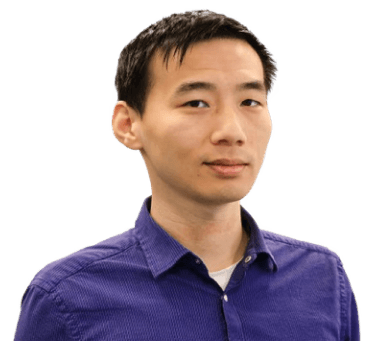
Aaron Li
Head of AI
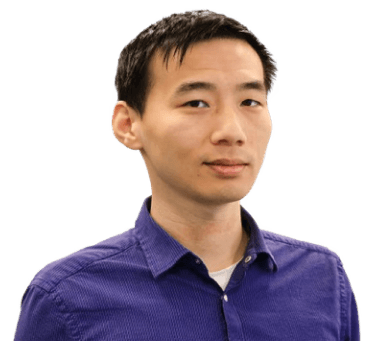
Aaron Li
Head of AI
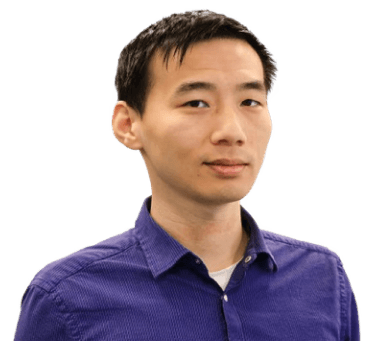
Liza Kharlova
Sr. Machine Learning Engineer
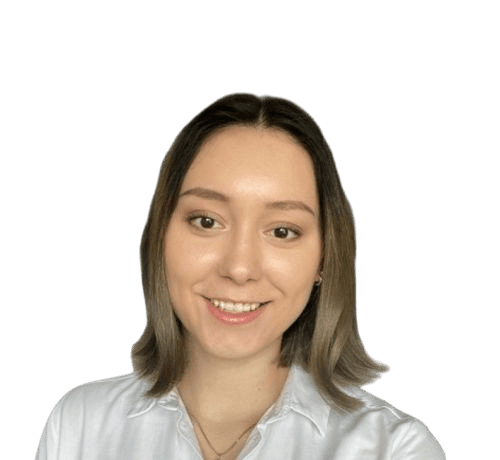
Liza Kharlova
Sr. Machine Learning Engineer
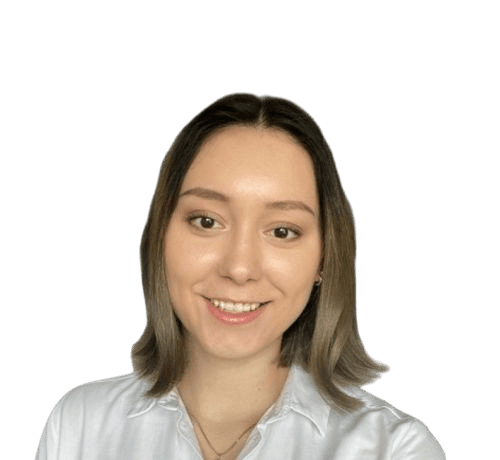
Arghya Kundu
Sr. Machine Learning Engineer
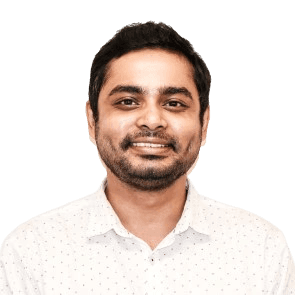
Arghya Kundu
Sr. Machine Learning Engineer
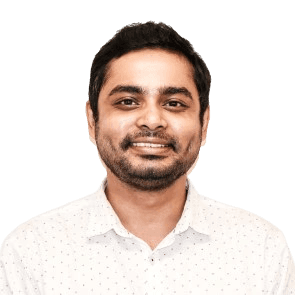
Rohan Aswani
Sr. Machine Learning Engineer
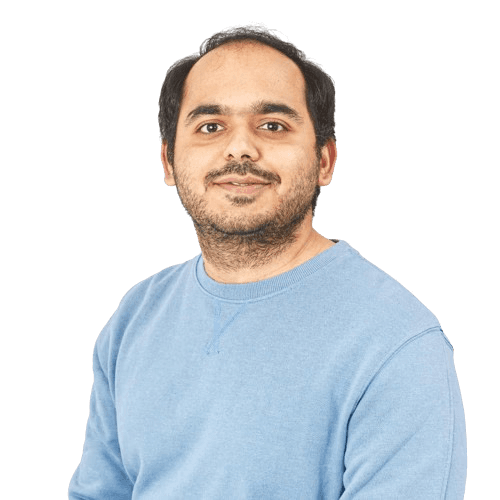
Rohan Aswani
Sr. Machine Learning Engineer
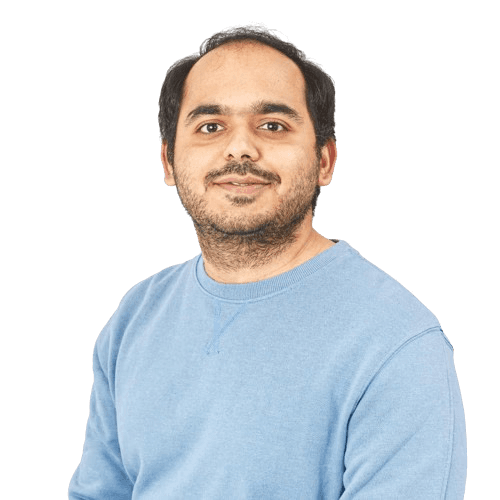
Liza Kharlova
Sr. Machine Learning Engineer
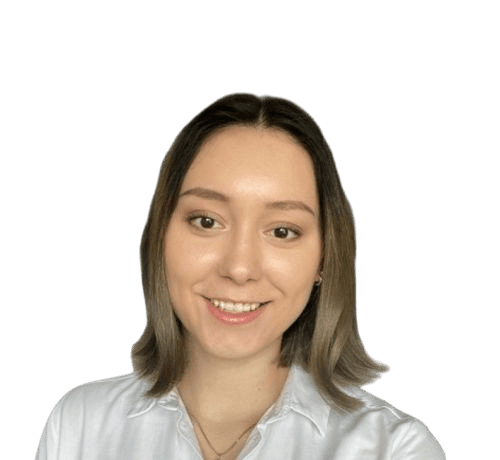
Liza Kharlova
Sr. Machine Learning Engineer
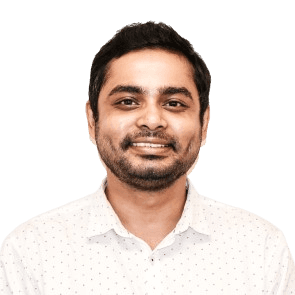
Arghya Kundu
Sr. Machine Learning Engineer
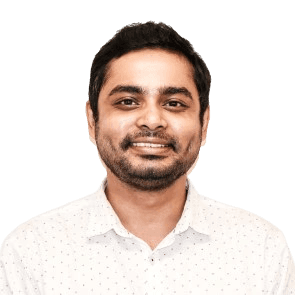
Rohan Aswani
Sr. Machine Learning Engineer
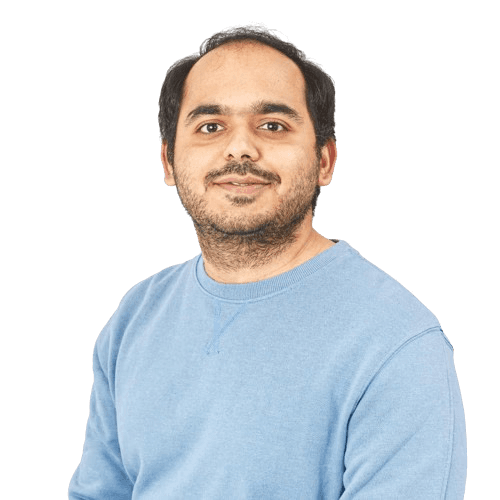
Liza Kharlova
Sr. Machine Learning Engineer
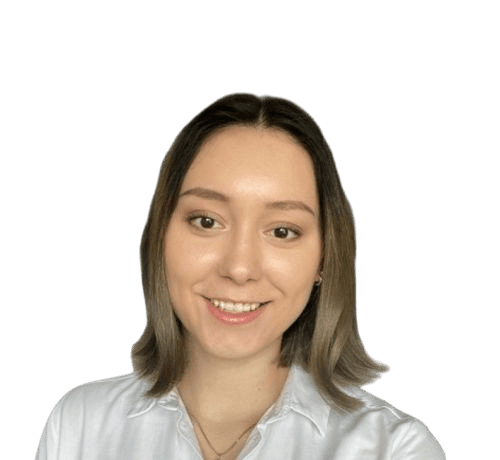
Liza Kharlova
Sr. Machine Learning Engineer
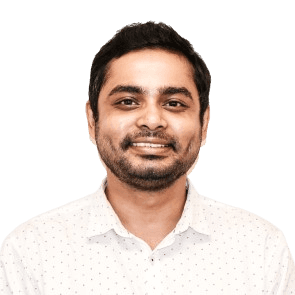
Arghya Kundu
Sr. Machine Learning Engineer
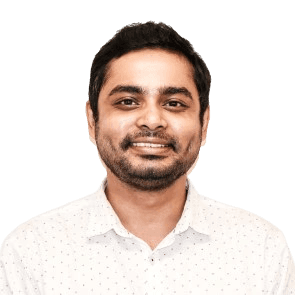
Rohan Aswani
Sr. Machine Learning Engineer
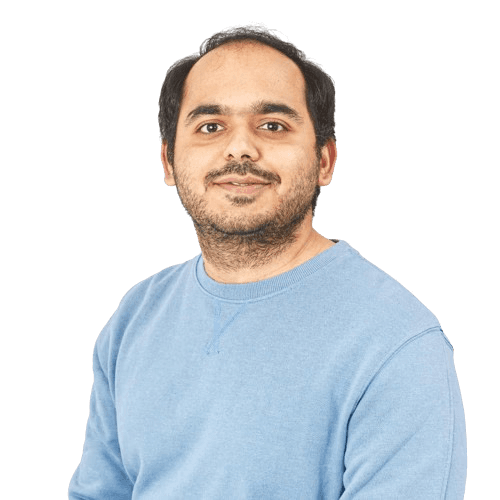
Matt Bogoroch
CEO and Co-founder
Ex-KPMG Data Science
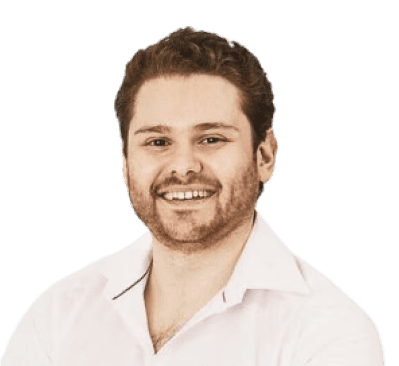
Matt Bogoroch
CEO and Co-founder
Ex-KPMG Data Science
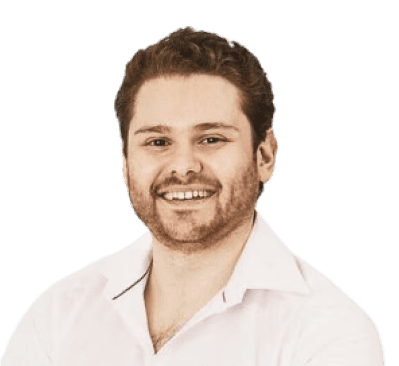
Shardul Frey
CEO and Co-founder
Specialty in Applied Mathematics
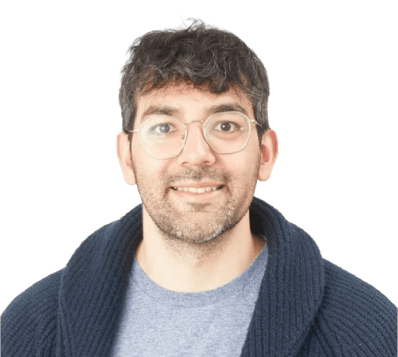
Shardul Frey
CEO and Co-founder
Specialty in Applied Mathematics
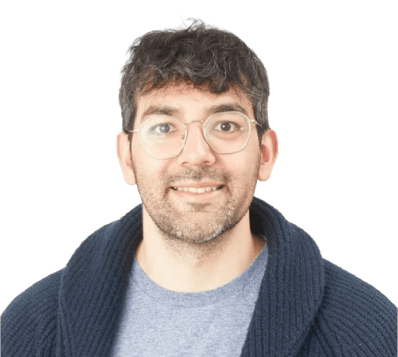
Aaron Li
Head of AI
Specialty in Machine Learning
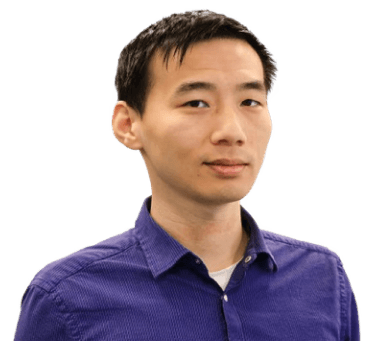
Aaron Li
Head of AI
Specialty in Machine Learning
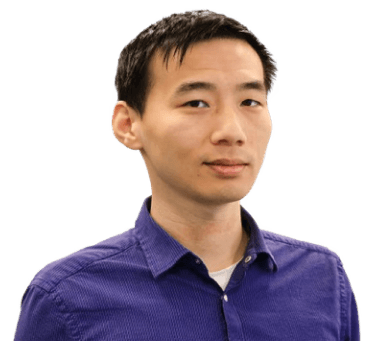
Liza Kharlova
Sr. ML Engineer
Specialty in Machine Learning
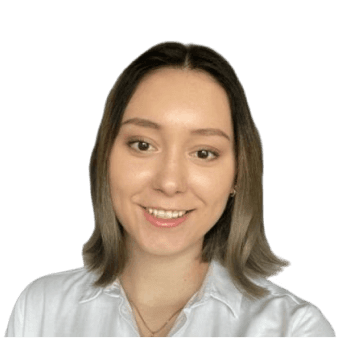
Liza Kharlova
Sr. ML Engineer
Specialty in Machine Learning
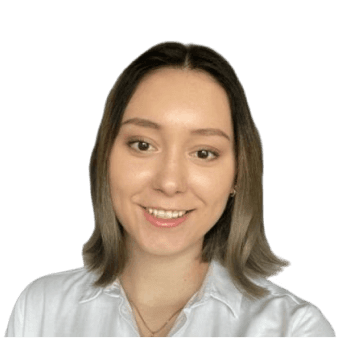
Pricing
Getting started is easy
Simple, transparent pricing with no long-term commitments.
BirdseyeQSR
What we offer
Reporting and insights through the Birdseye dashboard to measure sales lift, customer behavior and trends
Communicate on your terms — Ongoing meetings at your preferred cadence, task tracking portal, 24/7 support
Continuous optimization and refinement of the model as it learns from your data
Strategic guidance — Birdseye will assist you in developing a data roadmap and customer engagement strategy
Flexible and secure data integration via SFTP, API, or import - data stays on your tenant
Custom AI Model for your QSR, based on your unique menu items, loyalty programs, sales, and third-party data
Personalization Engine to deliver tailored offers to your customers across touch points
BirdseyeQSR
What we offer
Reporting and insights through the Birdseye dashboard to measure sales lift, customer behavior and trends
Communicate on your terms — Ongoing meetings at your preferred cadence, task tracking portal, 24/7 support
Continuous optimization and refinement of the model as it learns from your data
Strategic guidance — Birdseye will assist you in developing a data roadmap and customer engagement strategy
Flexible and secure data integration via SFTP, API, or import - data stays on your tenant
Custom AI Model for your QSR, based on your unique menu items, loyalty programs, sales, and third-party data
Personalization Engine to deliver tailored offers to your customers across touch points
BirdseyeQSR
What we offer
Reporting and insights through the Birdseye dashboard to measure sales lift, customer behavior and trends
Communicate on your terms — Ongoing meetings at your preferred cadence, task tracking portal, 24/7 support
Continuous optimization and refinement of the model as it learns from your data
Strategic guidance — Birdseye will assist you in developing a data roadmap and customer engagement strategy
Flexible and secure data integration via SFTP, API, or import - data stays on your tenant
Custom AI Model for your QSR, based on your unique menu items, loyalty programs, sales, and third-party data
Personalization Engine to deliver tailored offers to your customers across touch points
The Birdseye Difference —
Committed to your success
The Birdseye Difference
Seamless extension of your team
Birdseye provides proactive support and expertise that fill internal knowledge gaps.
Strategic guidance and support
We can help shape your analytics roadmap, identifying growth opportunities through advanced analytics.
Measurable results
Dedicated to delivering clear outcomes that boost customer loyalty and profitability.
Cancel anytime
Cancel anytime, no long-term commitment—restart whenever you're ready.
Unlock the power of your data with BirdseyeQSR
Get started,
it's free!
Offer diners exactly what they want, when they want it, so they spend more, dine more and efficiently grow your profits.
Connect Birdseye to your Shopify store and start sending personalized emails.
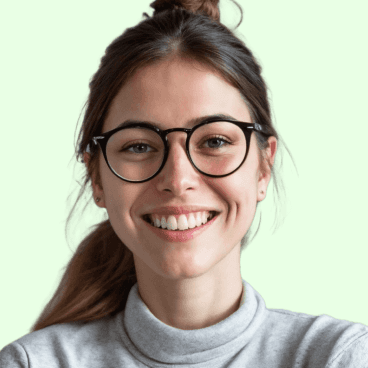
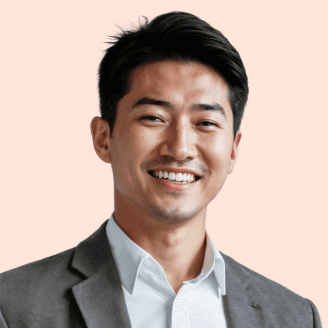
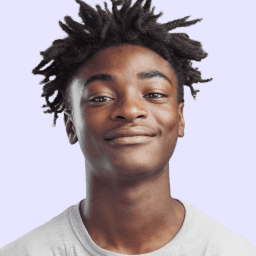
Eat. Sleep. Personalize. Repeat. Eat. Sleep. Personalize. Repeat. Eat. Sleep. Personalize. Repeat. Eat. Sleep. Personalize. Repeat. Eat. Sleep. Personalize. Repeat. Eat. Sleep. Personalize. Repeat. Eat. Sleep. Personalize. Repeat.